Population-based studies have estimated that half of the Hispanic patients with glaucoma1 and more than 90% of glaucoma patients in developing nations2,3 are undiagnosed until the disease reaches advanced stages or are unaware that they have the disease.
While this is partially due to the asymptomatic nature of the vision loss that delays patients in seeking care, the lack of a universal, reliable, inexpensive and widely available tool makes screening efforts for glaucoma even more challenging.
Although yet to reach clinical adoption, recent studies have shown that artificial intelligence (AI) — in particular, deep learning (DL) — can be used for the detection of glaucoma and can identify glaucomatous change more quickly and accurately than traditional methods.
THE PROMISE OF AI FOR GLAUCOMA
AI dates back to the 1950s,4 and was once perceived as a science-fiction theory or something far removed from reality. Knowledge was limited to selected groups of computer scientists and enthusiasts. However, with the advent of free AI-powered applications, such as ChatGPT, AI has become more palpable to more users — and has also found its way into ophthalmology.
The most relevant type of AI currently used in ophthalmology is DL, in which models use artificial neural networks, which consist of several layers that successively receive data (such as fundus images or visual field and optical coherence tomography [OCT] parameters, for example), perform computations, and generate an output that is transmitted to the next layer to yield a final classification (eg, disease vs no disease).
AI has been used in glaucoma as early as 1994, when neural networks were trained for the interpretation of standard automated perimetry (SAP).5 However, the recent outstanding progress in computational power has allowed researchers to develop DL models capable of processing a large amount of complex information and of producing better results than humans in some tasks. These models have been proven to be effective for image classification and recognition, accomplished by extracting features from images and learning to recognize patterns that may not even be perceived by the human eye. Recent studies demonstrate how DL models can detect glaucoma and identify glaucomatous changes more quickly and accurately than traditional methods.
SCREENING FOR GLAUCOMA WITH AI
The ideal tool
Screening large populations for a disease poses a challenge when its diagnosis relies on individual examination by specialists, as is the case in glaucoma. To overcome this challenge, AI has emerged as a potential automated and efficient solution to identify diseases accurately without requiring a clinician.
For glaucoma screening, the most promising application of AI is in the analysis of optic disc photographs. Optic disc photographs present the ideal screening tool for glaucoma due to their wide availability and low cost, with several portable fundus cameras that can potentially be easily used in low-resource settings.
By leveraging DL for the automated analysis of images, there is potential to extend the capabilities of fundus photographs to a much wider range of eye-care professionals, including those without specialized training. This could lead to more accurate and accessible diagnoses.
Smoothing out the kinks
In 2017, Ting and colleagues developed a DL algorithm trained to identify glaucomatous changes in fundus photos.6 Using a large database of 494,661 fundus photographs from diabetes patients, subjectively classified by professional graders from a teleophthalmology program, the authors developed an algorithm capable of detecting images that were considered “referable” or not for glaucoma. In the test dataset, their algorithm detected “referable” glaucoma on photographs with an area under the receiver operating characteristic (AUC) curve of 0.942.
However, at the level of specificity reported (87.2%), their approach would result in a high number of healthy individuals being unnecessarily referred for evaluation (ie, false positives). This would be a problem, potentially leading to a surge of unnecessary referrals that could overwhelm health-care resources and place a burden on patients.7
In a similar approach, Liu et al used 48,116 color fundus images to detect “referable” glaucoma based on human gradings, achieving an AUC of 0.986 and a higher specificity of 92%.8 However, relying on subjective gradings to train DL models is problematic. Overall, subjective gradings tend to have low reproducibility and poor agreement, even when completed by experts.9 When subjective gradings are used as the reference standard to train a DL model, the trained model will carry forward these imperfections.
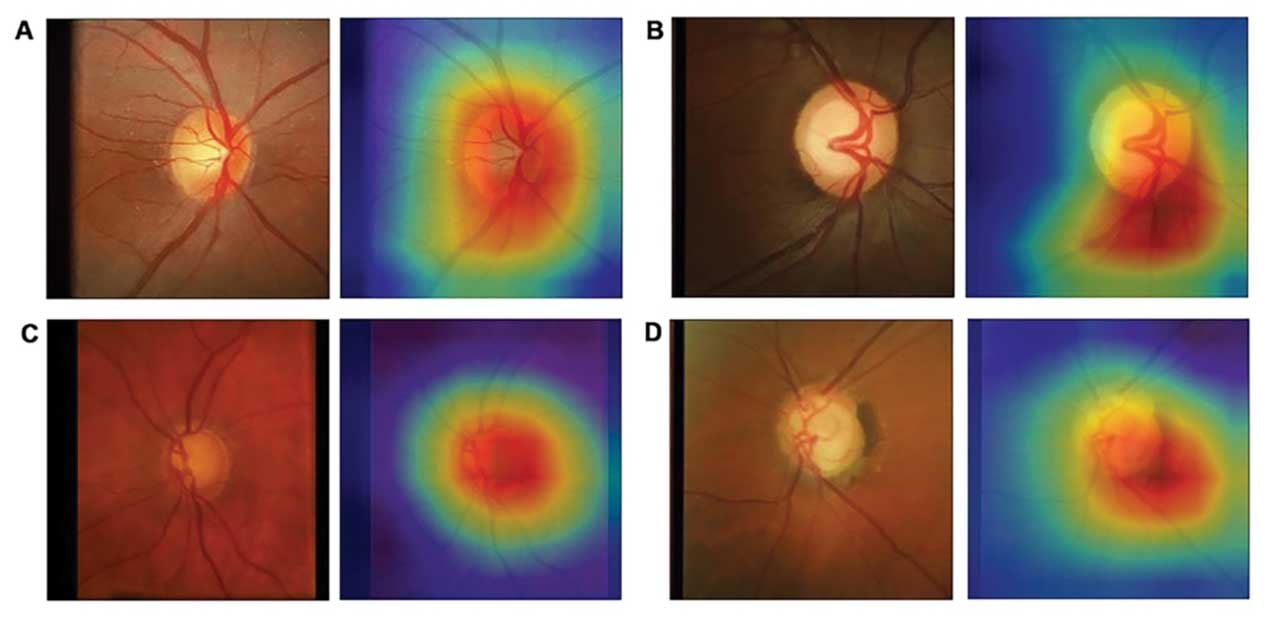
Success with machine to machine
To bypass subjective grading, Medeiros et al developed a DL model to quantify retinal nerve fiber layer (RNFL) thickness from fundus photographs (Figure).10 Instead of human gradings, the photos were labeled with objective global RNFL thickness measurements from spectral-domain OCT in a “machine to machine” (M2M) approach. The authors demonstrated a strong correlation between the predicted RNFL value from the photo-based DL algorithm and the actual RNFL thickness value from the corresponding OCT (r=0.832, p<0.001) with a mean absolute error of only 7 µm, similar to the variability of the actual OCT machine.
In a subsequent investigation, the M2M RNFL predictions performed better than subjective human gradings to detect glaucomatous damage.11 With a quantifiable and objective metric, this approach can derive cut-offs to achieve desired specificity levels in order to optimize its application in a screening setting.
AI IN GLAUCOMA PROGRESSIONS
Recent studies using DL have shown promising results in identifying glaucoma progression from fundus photos, OCT and SAP data. In the first application of DL to detect glaucoma progression from fundus photos, the M2M algorithm was able to detect relatively small changes in the optic disc over time that were highly correlated to actual changes in RNFL thickness over time.12
The model was also useful in assessing the risk of future development of visual field loss in eyes suspected of glaucoma.13 Wen and colleagues utilized a DL model to generate a “point-wise visual field prediction” from a large database of SAP tests, outperforming linear models in predicting future visual fields in glaucomatous eyes up to 5.5 years in advance.14
For OCT, Mariottoni et al published a model that was able to assess the presence of glaucoma progression as well as pinpoint the location of regions of likely progression on RNFL thickness measurements.15 The model agreed well with expert judgments of progression and proved to be a significant improvement over conventional trend-based analyses of progression.
In a study using OCT to predict progression on SAP, Hou used a new state-of-the-art DL approach called Gated Transformer Network that was trained and optimized with a consensus-based definition of glaucoma based on visual field parameters.16 When applied to a longitudinal series of OCT imaging, the model was able to predict visual field loss with an AUC of 0.97. By using OCT images to detect clinically significant visual field deterioration, this approach may reduce the need to rely on SAP testing.
Overall, the integration of DL algorithms in glaucoma progression detection shows great promise, but further research is needed to validate their performance both over longer periods and in diverse patient populations.
REMAINING CHALLENGES
The introduction of AI into the medical field presents a transformative opportunity for the assessment of medical images. Studies have demonstrated how AI can be used to successfully identify glaucomatous damage in fundus images and patterns of RNFL visual field loss over time. However, several challenges need to be addressed before AI can be successfully deployed in glaucoma.
For comparison, while the FDA approved the first AI diagnostic platform for diabetic retinopathy in 2018, the lack of consistent objective diagnostic criteria for glaucoma has made it particularly difficult to approve any algorithm for glaucoma screening. The findings associated with glaucoma are considerably more challenging to identify than diabetic retinopathy due to the wide range of optic disc presentations in the population and the lack of a universal consensus definition for glaucomatous optic neuropathy.7 Most models have yet to reach interpretability and health economic analysis justification, which are hindered by ethical, medico-legal and logistical barriers.17 However, numerous AI algorithms are in the works for glaucoma that have the potential to completely transform glaucoma care in the near future. OM
References
- Quigley HA, West SK, Rodriguez J, et al. The prevalence of glaucoma in a population-based study of Hispanic subjects: Proyecto VER. Arch Ophthalmol 2001;119:1819-1826.
- Budenz DL, Barton K, Whiteside-de Vos J, et al. Prevalence of glaucoma in an urban West African population: the Tema Eye Survey. JAMA Ophthalmol 2013;131:651-658.
- Hennis A, Wu SY, Nemesure B, et al. Awareness of incident open-angle glaucoma in a population study: the Barbados Eye Studies. Ophthalmology 2007;114:1816-1821.
- Turing AM. Computing machinery and intelligence. Mind 1950;59(236):433.
- Goldbaum MH, Sample PA, White H, et al. Interpretation of automated perimetry for glaucoma by neural network. Invest Ophthalmol Vis Sci 1994;35:3362-3373.
- Ting DSW, Cheung CY, Lim G, et al. Development and Validation of a Deep Learning System for Diabetic Retinopathy and Related Eye Diseases Using Retinal Images From Multiethnic Populations With Diabetes. JAMA 2017;318:2211-2223.
- Medeiros FA, Lee T, Jammal AA, et al. The Definition of Glaucomatous Optic Neuropathy in Artificial Intelligence Research and Clinical Applications. Ophthalmol Glaucoma 2023;6:432-438.
- Li Z, He Y, Keel S, et al. Efficacy of a Deep Learning System for Detecting Glaucomatous Optic Neuropathy Based on Color Fundus Photographs. Ophthalmology 2018;125:1199-1206.
- Varma R, Steinmann WC, Scott IU. Expert agreement in evaluating the optic disc for glaucoma. Ophthalmology 1992;99:215-221.
- Medeiros FA, Jammal AA, Thompson AC. From Machine to Machine: An OCT-Trained Deep Learning Algorithm for Objective Quantification of Glaucomatous Damage in Fundus Photographs. Ophthalmology 2019;126:513-521.
- Jammal AA, Mariottoni E, Thompson A, et al. Human versus Machine: Comparing a Deep Learning Algorithm to Human Gradings for Detecting Glaucoma on Fundus Photographs. Am J Ophthalmol (in press).
- Medeiros FA, Jammal AA, Mariottoni EB. Detection of Progressive Glaucomatous Optic Nerve Damage on Fundus Photographs with Deep Learning. Ophthalmology 2021;128:383-392.
- Lee T, Jammal AA, Mariottoni EB, Medeiros FA. Predicting Glaucoma Development With Longitudinal Deep Learning Predictions From Fundus Photographs. Am J Ophthalmol 2021;225:86-94.
- Wen JC, Lee CS, Keane PA, et al. Forecasting future Humphrey Visual Fields using deep learning. PLoS One 2019;14:e0214875.
- Mariottoni EB, Datta S, Shigueoka LS, et al. Deep Learning-Assisted Detection of Glaucoma Progression in Spectral-Domain OCT. Ophthalmol Glaucoma 2023;6:228-238.
- Hou K, Bradley C, Herbert P, et al. Predicting Visual Field Worsening with Longitudinal OCT Data Using a Gated Transformer Network. Ophthalmology 2023;130:854-862.
- Correia Barao R, Hemelings R, Abegao Pinto L, et al. Artificial intelligence for glaucoma: state of the art and future perspectives. Curr Opin Ophthalmol 2023. Epub 2023 Nov. 29.