Machine learning (ML) is a field of artificial intelligence that enables systems to learn and improve from experience without being explicitly programmed, allowing them to automatically discover patterns and make decisions or predictions based on data.
The integration of ML into medicine and ophthalmology represents a significant turning point in health care. The application of advanced algorithms and artificial intelligence has the potential to reshape the way we approach diagnosis, treatment and patient care.1,2 As we stand at the intersection of technology and health care, it is vital for health-care professionals, researchers and the general public to grasp the significance of this transformative shift.
This article aims to shed light on the advantages and drawbacks of harnessing ML in the medical and ophthalmological domains, offering a nuanced perspective on its impact and the crucial reasons to engage with this topic. The importance of this discussion lies in its potential to drive informed decision-making, policy development and ethical considerations in the health-care industry. With ML increasingly becoming a fundamental tool, understanding its implications is crucial for ensuring the responsible and effective implementation of these technologies. By exploring the pros and cons of ML in medicine and ophthalmology, we aim to provide the knowledge required to navigate the evolving landscape of health care, fostering a deeper appreciation for the opportunities and challenges it presents.
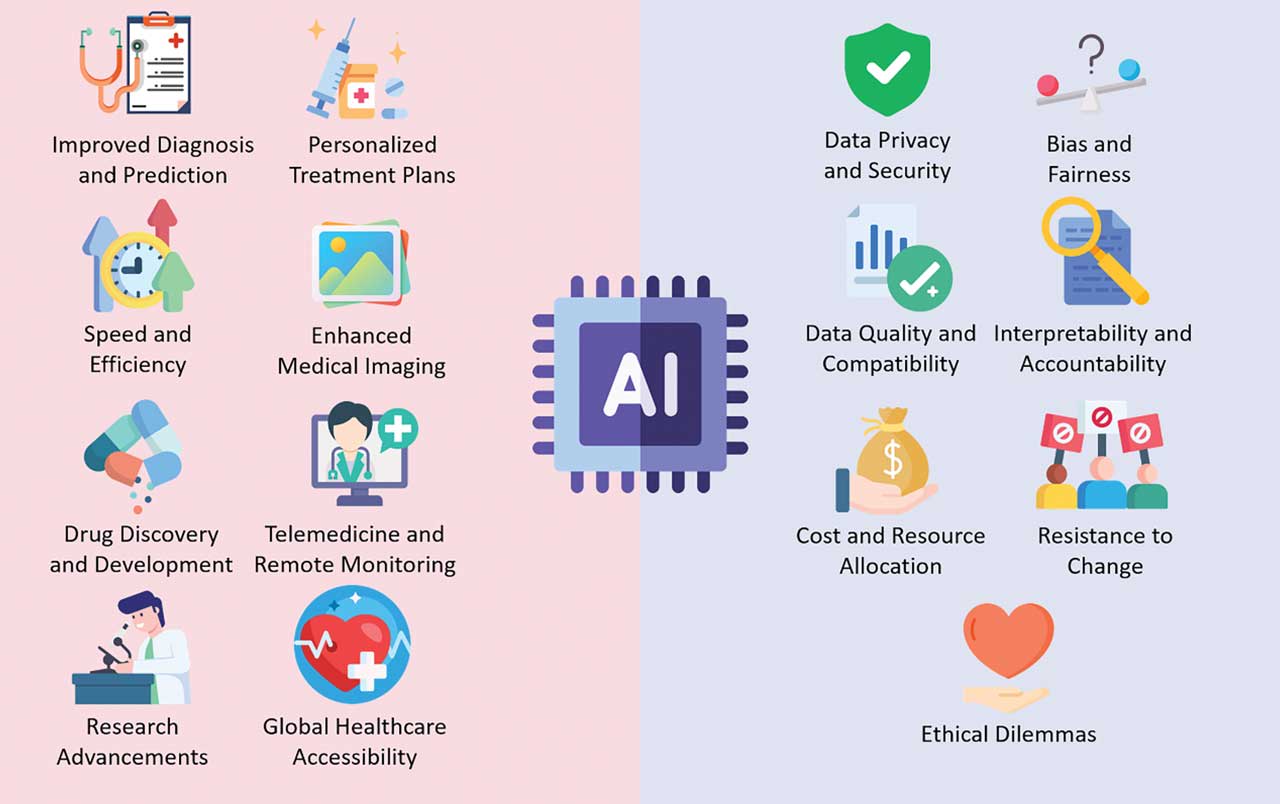
Pros of Machine Learning
Improved diagnosis and prediction
ML algorithms excel at pattern recognition, enabling them to analyze vast amounts of medical data to assist in diagnosing diseases and predicting patient outcomes. This leads to more accurate and timely diagnoses, reducing the risk of missed or delayed diagnoses and improving patient prognosis.3
For instance, in ophthalmology, ML models can identify subtle changes in retinal images to detect eye diseases like diabetic retinopathy at an early stage, allowing for prompt treatment and preserving vision.
Personalized treatment plans
The ability of ML to process large datasets on patient histories and genetics allows for the development of personalized treatment plans. These plans can factor in a patient’s unique genetic makeup and medical history to determine the most effective course of action.
In ophthalmology, ML can assist in diagnosing eye diseases by analyzing retinal scans or images. It can predict conditions such as diabetic retinopathy or AMD, aiding in early detection and personalized treatment plans for patients, thereby potentially preserving vision and preventing irreversible damage.
Speed and efficiency
Automation of tasks within health-care systems can significantly enhance efficiency. Administrative tasks, such as appointment scheduling and medical billing, can be streamlined, reducing the workload on health-care staff and minimizing errors.
Additionally, ML algorithms can streamline patient triage by analyzing various factors such as vital signs, medical history and reported symptoms. These algorithms can swiftly identify patients at higher risk or with urgent medical needs, aiding health-care providers in prioritizing care and allocating resources effectively. The efficiency gains are particularly critical in reducing health-care costs and improving the patient experience.
Enhanced medical imaging
ML algorithms can process medical images with exceptional precision. In ophthalmology, this means accurate and rapid analysis of retinal scans, allowing for early detection and monitoring of conditions like glaucoma and macular degeneration. In radiology, ML can enhance the detection of abnormalities in X-rays, CT scans and MRIs, enabling quicker diagnoses and potentially life-saving interventions.
Drug discovery and development
ML can expedite the drug discovery process. By analyzing massive datasets, it can identify potential drug candidates, predict their effectiveness and optimize the development process. This can significantly reduce the time and resources required to bring new medications to market.
In ophthalmology, this could lead to the discovery of novel treatments for conditions like AMD or glaucoma.
Telemedicine and remote monitoring
The capabilities of ML enable telemedicine and remote patient monitoring to thrive. Remote patient monitoring devices equipped with machine learning algorithms can continuously analyze patient data, such as vital signs or blood glucose levels, and alert health-care
providers to changes in a patient’s condition.
This is especially beneficial for patients with chronic diseases, including those related to ophthalmology, allowing them to receive timely interventions and reducing the burden on health-care facilities.
Research advancements
ML can accelerate medical research by processing vast datasets, identifying patterns and generating hypotheses. This can lead to breakthroughs in understanding diseases and developing innovative treatments.
In ophthalmology, for example, ML aids in understanding the underlying causes of various eye conditions and can contribute to the development of novel treatment strategies.
Global health-care accessibility
ML can help bridge the gap in health-care accessibility between regions with limited resources and those with advanced health-care systems. Telemedicine and remote monitoring, powered by ML, enable expert care to reach underserved populations, including remote areas and low-resource communities. It does so by leveraging data analytics to recognize patterns, predict health outcomes and optimize care plans based on individual patient data.
Compared to methods not using ML, this approach allows for more precise and targeted interventions, especially beneficial in areas with limited access to healthcare resources, resulting in improved health-care outcomes for underserved populations.
Cons of Machine Learning
Data privacy and security
The vast amount of patient data required for ML applications raises significant concerns about data privacy and security. Health-care organizations must invest in robust security measures to protect sensitive medical information from breaches and unauthorized access.4 Data privacy regulations, like HIPAA, further complicate the use of patient data for ML while ensuring patient rights are respected.5
Bias and fairness
ML models are only as good as the data they are trained on. If the data used is biased or unrepresentative, it can lead to disparities in health-care outcomes. Biases in data can disproportionately affect certain patient groups, potentially discriminating against them in diagnosis, treatment or access to health-care services.6,7
In ophthalmology, an example of bias in health-care data could be related to retinal imaging. If the dataset used to train an AI system for diagnosing eye diseases primarily consists of images from a specific demographic, such as a particular ethnic group or age range, the model might not generalize well to other demographics. This bias could lead to less accurate diagnoses or missed detections for individuals whose data wasn’t well-represented in the training dataset, potentially affecting their access to appropriate treatments or interventions. Ensuring data quality and addressing biases is an ongoing challenge.
Data quality and compatibility
ML relies heavily on high-quality and interoperable data. Inconsistent or incomplete medical records and data incompatibility between different systems can hinder the effectiveness of machine learning applications. Data quality issues can lead to inaccuracies and misinterpretations in diagnoses and treatment recommendations.
Interpretability and accountability
The “black-box” nature of some machine learning algorithms can make it difficult to understand their decision-making processes.8 This lack of transparency can create challenges in holding both the algorithms and health-care providers accountable for their actions. Patients and health-care professionals may be skeptical of machine-generated recommendations if they cannot understand the rationale behind them.
Cost and resource allocation
Implementing ML in health care demands substantial financial investments. Hospitals and clinics need to purchase advanced equipment, acquire software licenses and provide training for staff. This allocation of resources may divert funding from other critical areas of health care, and it can be especially challenging for smaller facilities with limited budgets.
Resistance to change
Health-care professionals are often accustomed to traditional workflows and may be resistant to adopting new technology, particularly if it disrupts established practices and necessitates extensive training.9 Overcoming this resistance to change and ensuring that health-care providers are comfortable with ML tools can be a significant hurdle in the adoption of this technology.
Ethical dilemmas
The integration of ML in medicine and ophthalmology raises several ethical dilemmas. Health-care practitioners and institutions must grapple with questions like when to trust machine recommendations over human judgment, how to balance cost-effectiveness with patient care and whether machine-assisted health care will depersonalize patient interactions.10 Ethical considerations are crucial to ensure that patient well-being remains the primary focus.11
Conclusion
The integration of ML in medicine and ophthalmology is a profound transformation in health care, with far-reaching implications. Its advantages, such as improved diagnosis, personalized treatment and enhanced efficiency, hold the promise of better patient care and more efficient health-care systems. However, the cons, including data privacy concerns, bias, accountability challenges and ethical dilemmas, underscore the need for careful and responsible implementation.
This technology is not without its complexities, but it is already making a substantial impact on health care. The responsible use of ML in medicine and ophthalmology is essential, and the continuous exploration of its pros and cons is fundamental in achieving a balance between technological innovation and ethical patient care.
As we move forward, health-care professionals, policymakers and researchers must collaborate to ensure that ML serves as a tool for the betterment of health care, ultimately improving patient outcomes and promoting a more sustainable and effective health-care system. By understanding the advantages and drawbacks of this technology, we can navigate the evolving landscape of health care with wisdom, innovation and a commitment to patient well-being. OM
References
1. Chen PC, Liu Y, Peng L. How to develop machine learning models for healthcare. Nature materials. 2019;18(5):410-414.
2. Ting DSW, Peng L, Varadarajan AV, Keane PA, Burlina PM, Chiang MF, et al. Deep learning in ophthalmology: The technical and clinical considerations. Progress in retinal and eye research. 2019;72:100759.
3. Topol EJ. High-performance medicine: the convergence of human and artificial intelligence. Nature medicine. 2019;25(1):44-56.
4. Zhou Q, Zuley M, Guo Y, Yang L, Nair B, Vargo A, et al. A machine and human reader study on AI diagnosis model safety under attacks of adversarial images. 2021;12(1):7281.
5. Tom E, Keane PA, Blazes M, Pasquale LR, Chiang MF, Lee AY, et al. Protecting Data Privacy in the Age of AI-Enabled Ophthalmology. Translational vision science & technology. 2020;9(2):36.
6. Wu E, Wu K, Daneshjou R. How medical AI devices are evaluated: limitations and recommendations from an analysis of FDA approvals. 2021;27(4):582-584.
7. Gianfrancesco MA, Tamang S, Yazdany J, Schmajuk G. Potential Biases in Machine Learning Algorithms Using Electronic Health Record Data. JAMA internal medicine. 2018;178(11):1544-1547.
8. Funer F. The Deception of Certainty: how Non-Interpretable Machine Learning Outcomes Challenge the Epistemic Authority of Physicians. A deliberative-relational Approach. Medicine, health care, and philosophy. 2022;25(2):167-178.
9. González-Gonzalo C, Thee EF, Klaver CCW, Lee AY, Schlingemann RO, Tufail A, et al. Trustworthy AI: Closing the gap between development and integration of AI systems in ophthalmic practice. Progress in retinal and eye research. 2021:101034.
10. Grote T, Berens P. On the ethics of algorithmic decision-making in healthcare. Journal of medical ethics. 2020;46(3):205-211.
11. Abdullah YI, Schuman JS, Shabsigh R, Caplan A, Al-Aswad LA. Ethics of Artificial Intelligence in Medicine and Ophthalmology. Asia-Pacific journal of ophthalmology (Philadelphia, Pa). 2021;10(3):289-298.