With the onslaught of information predicting how artificial intelligence (AI) will some day transform medicine, clinicians can lose focus on how AI is helping in the real world right now. One area in which AI is making a present-day difference, and will likely make significant contributions in the future, is in diagnosing disease.
In this article, we’ll look at the way AI is being used for diagnosis today, the drivers behind the use of AI and explore how AI may help in diagnostics in the future.
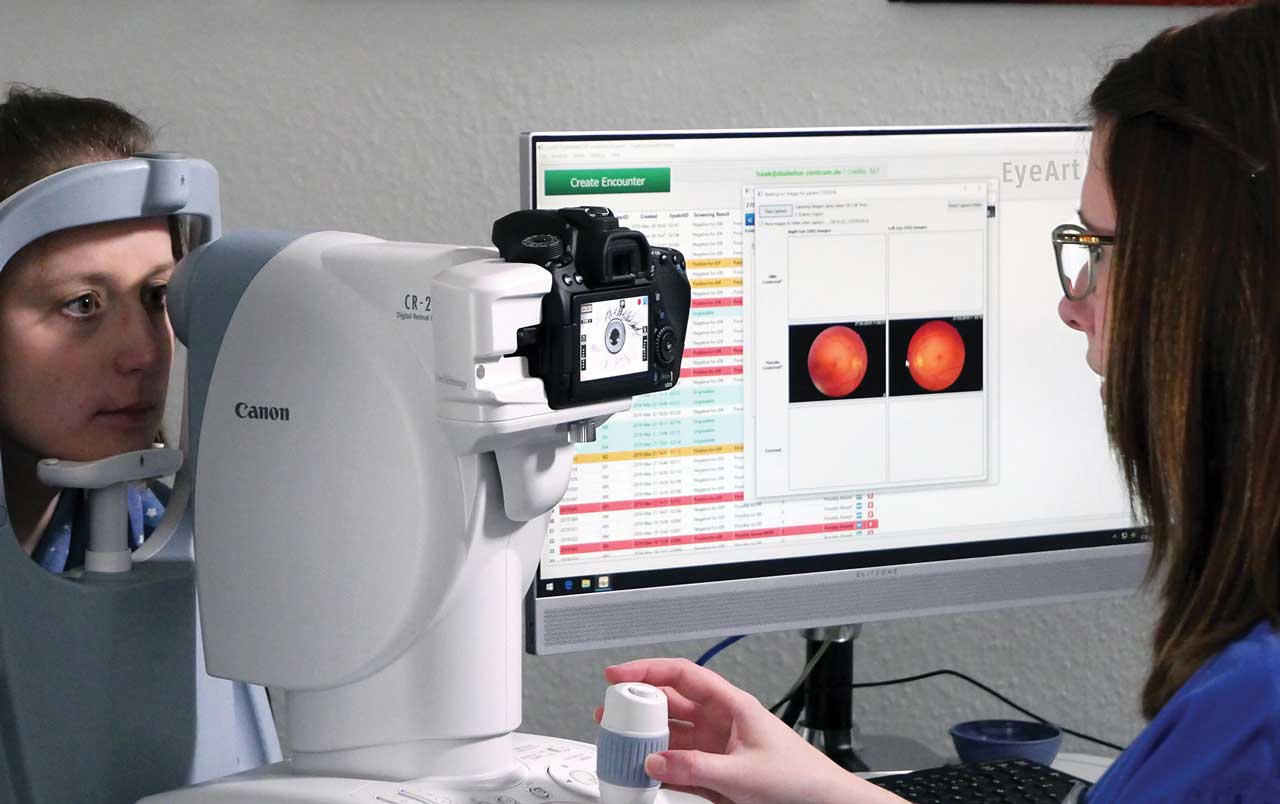
The current landscape
Today, the main use of AI for diagnosis in ophthalmology involves screening patients for diabetic retinopathy (DR). The FDA has approved three devices that use AI to diagnose DR: the EyeArt system (Eyenuk); AEYE-DS (AEYE Health); and Luminetics-Core (Digital Diagnostics), formerly known as IDx-DR.These systems are intended to be used not in ophthalmology offices, but in primary care. The goal is quick diagnosis of patients with signs of DR so that they can be referred to an ophthalmologist for further evaluation and treatment.
“In so many places where people have limited access to care or where they’re just not going to get to care, if you can get an image of the eye, then you can at least categorize their risk, and that’s very useful,” says John A. Hovanesian, MD, Harvard Eye Associates, Laguna Hills, Calif.
This use of AI represents a “great kind of initial use of AI in ophthalmology and in medicine,” says Theodore Leng, MD, MS, associate professor of ophthalmology and, by courtesy, of anesthesiology, perioperative and pain medicine, Stanford Byers Eye Institute, Stanford Medicine.
Ophthalmology on the cutting edge
Arguably, ophthalmology is at the forefront of AI in medicine. The LumineticsCore “was the first FDA-cleared device to autonomously diagnose anything in any field of medicine,” says Grayson W. Armstrong, MD, MPH, director, ophthalmology emergency services, Massachusetts Eye & Ear, Harvard Medical School, and a cataract surgeon and retina specialist.
What’s more, the use of AI devices to detect DR carries a CPT code (92229, original effective date September 2021) facilitating reimbursement. “For the first time, we’ve proven that there’s a reimbursement pathway to make AI reimbursable and self-sustaining in the clinic,” Dr. Armstrong says.
“You cannot buy a fully autonomous, self-driving car that is street-legal, but you can get diagnosed by a ‘street-legal,’ FDA-cleared, bioethically sound, reimbursable autonomous AI,” adds Michael Abramoff, MD, PhD, founder and executive chairman, Digital Diagnostics.
Two drivers
Two factors are driving the use of AI to diagnose DR, according to Dr. Leng. First, the screening rate for patients with diabetes is low, and increasing the screening rate will address a “huge unmet need in medicine and society,” says Dr. Leng.
Second, he says, training the algorithms used for DR is relatively straightforward. Essentially, the algorithms look for the presence of blood in two-dimensional color photographs, he says. Today, he notes, multiple algorithms for this purpose have been published or presented at conferences.
In the case of EyeArt, for instance, Eyenuk developers trained the system on hundreds of thousands of images and developed “explainability modules” that articulate why the system suspects disease, according to Kaushal Solanki, PhD, Eyenuk CEO and founder. The EyeArt system, according to Eyenuk, is the only AI system cleared by the FDA for detecting both more-than-mild DR and vision-threatening DR and for use with multiple retinal cameras by different manufacturers. It first received FDA clearance in August 2020.
In April 2018, Digital Diagnostics announced that the FDA granted the company’s De Novo request to market LumineticsCore. The device, according to the firm, was the first autonomous, AI-based diagnostic system authorized for commercialization by the FDA. On November 2022, the FDA cleared the use of AEYE-DS for the detection of more-than-mild DR with the Topcon NW400 camera.
Dr. Leng notes that the FDA approves these systems based on a particular algorithm; if the system is changed, then it must go through another FDA approval process.
Future uses
In the future, notes Dr. Leng, AI will move beyond diabetic retinopathy to “layering on more diagnoses.” AI algorithms are likely to help diagnose AMD and glaucoma, and a single AI-enabled system could potentially diagnose all of these conditions. In addition, AI might help with screening patients in the ophthalmology office before the patient sees the physician. “I think the ability to have a machine pre-screen and look at all the pictures ahead of the patient encounter, and then augment our diagnostic capability, is also coming,” says Dr. Leng.
For glaucoma, no FDA-approved diagnostic device is available in the United States today, notes Arsham Sheybani, MD, associate professor of ophthalmology and visual sciences; residency program director and glaucoma fellowship director, Washington University School of Medicine, St. Louis. “There is a chance in the future that you’ll probably have an AI-driven system that looks at the field, the OCT, and then from there predicts who’s at higher risk for progression,” says Dr. Sheybani. He says he would be surprised “if in 10 years we weren’t using AI to help make decisions based on at least the imaging and the visual field testing for patients in glaucoma.”
In fact, a 2019 study by Liu et al comparing a deep-learning AI system with professional graders in detecting glaucomatous optic neuropathy found that the deep-learning system showed a sensitivity and specificity of greater than 90% for detecting the condition.
Beyond eye disease, Dr. Armstrong offers a larger view of how AI can work with fundus photography. Fundus photos and OCT, he notes, can offer a great deal of information about systemic health.
“I can imagine a future down the road where a fundus photograph or OCT can replace much of your lab work,” he says, adding that studies have shown fundus photos and OCT can identify evidence of conditions such as kidney disease, multiple sclerosis and Alzheimer’s.
“You can offload some of the invasive bloodwork and more extensive systemic testing and try to get that information purely from a picture of the back of the eye,” Dr. Armstrong says.
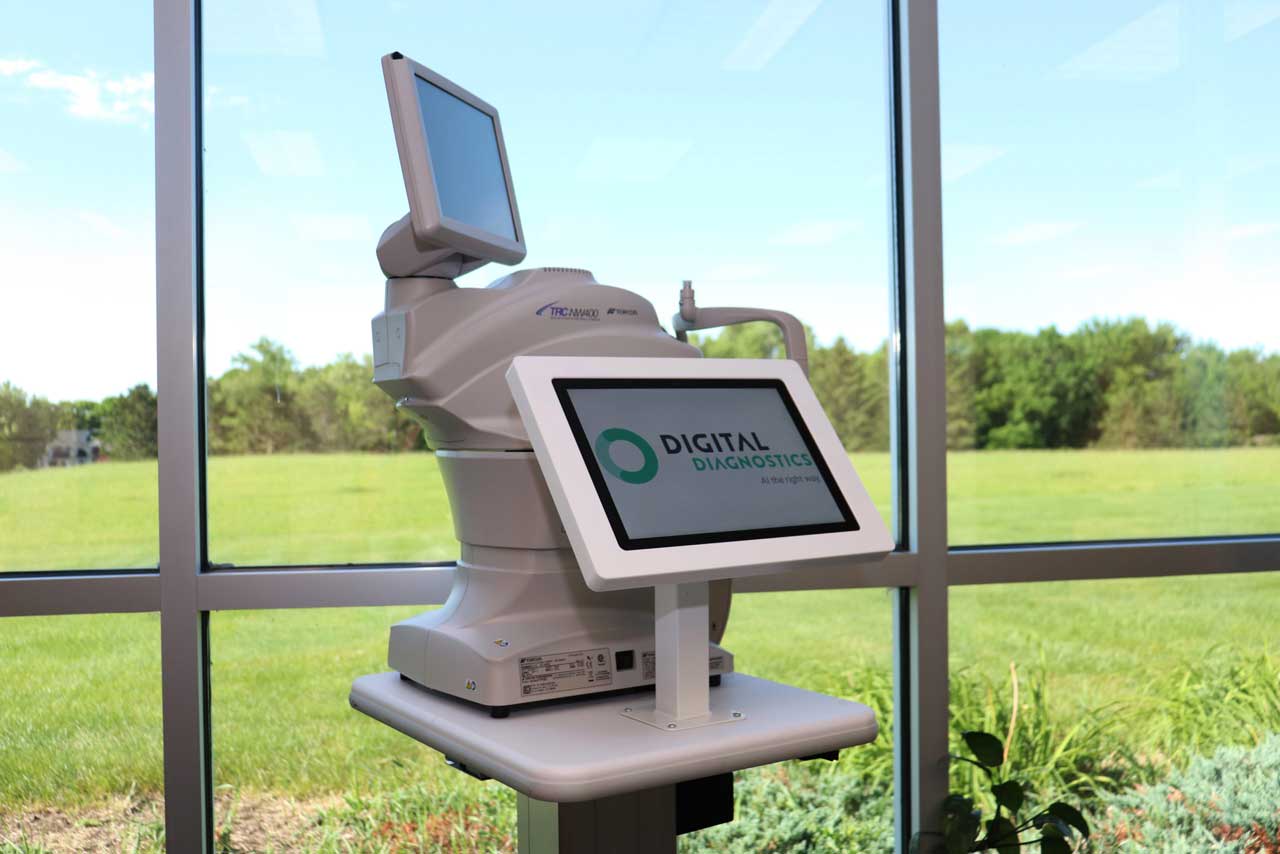
Tracking trends
Prognostication
One area where AI may provide significant benefit involves tracking trends and determining a patient’s prognosis.
An AI-enabled system, Dr. Leng notes, could potentially detect a subtle change in disease progression over time and alert the physician to investigate further. “I think a better use actually in the clinic is not for initial diagnosis for patients but more longitudinal management,” he says.
“I think the biggest benefit to ophthalmologists in the clinic is going to be prognostication,” says Dr. Armstrong.
He notes that an AI system could help answer questions such as: What is this patient’s potential vision a year down the road? What is this patient needing in terms of treatment? Might they benefit from an injection or not? Would this patient benefit from surgery?
“Those are the types of questions that people are asking and that AI is starting to answer,” Dr. Armstrong explains. “If you see them day one, perhaps the AI can give you a glimpse into the future of how it thinks things might go, which can help you in that moment know how aggressive or how intensive your therapy or surgery should be.”
Right now, Dr. Armstrong says, if he sees someone with glaucoma, he can give them statistics on how many patients on average will lose vision over the next 10 years. “But if I have an algorithm that can tell me for that specific patient, what their risk is with and without drops and with and without surgery, that’s much more targeted and much more personalized. It makes it a more directed, personalized approach.”
Three areas that will benefit
Dr. Abramoff looks at the future and predicts that AI will help in three broad areas. The first involves today’s diagnostic technology for DR, which will scale up to diagnose conditions such as glaucoma and AMD. The second is AI assisting the clinician, where there is interaction between the clinician and AI technology. While many might assume this will be an improvement, “in my view, we need to prove that,” Dr. Abramoff says. And third is the use of AI for research and exploratory work.
Challenges to overcome
When it comes to placing these devices for diagnosing DR in primary care offices, three challenges have to be met, notes Dr. Armstrong. The first involves the workflow challenge of placing AI in a primary care office. Making the devices user-friendly for staff that do not specialize in eye care is essential.
“Asking a primary care office to take a photo of every diabetic’s eye adds maybe 2 to 5 minutes per patient,” says Dr. Armstrong. “That adds up and it’s taking that nurse or that technician or that doctor away from doing something else.”
Making sure an efficient workflow is set up for identifying patients with diabetes needing imaging as well as capturing fundus photographs is critical.
A second challenge involves ensuring that the fundus photographs that were used to train the system do not lead to the device giving biased results. If the system was trained on patient populations such as mainly Caucasians, it may not do a great job of picking up disease, or even give inaccurate results, in African-American or Asian patients, he notes.
The third targets medico-legal issues, according to Dr. Armstrong. What are the legal ramifications if the AI-enabled device fails to pick up a disease that it was meant to discover? Does the fault lie with the manufacturer or the physician? And what happens if the photos show a dangerous condition such as a tumor that is not picked up because the algorithm is not trained for detecting such a disease?
Better together
Health care is notoriously slow to change, and it’s likely that some physicians will bring a good deal of skepticism to the use of AI for diagnosis. Dr. Hovanesian draws a parallel between the adoption of Goldmann visual fields and automated fields. While in the early days there was a question as to which was more accurate in gauging glaucoma progression, today no one uses Goldmann fields, he notes. The automated testing is likely more accurate than the Goldmann test, less time-consuming and requires less training to use.
“I think that data is going to show over time that we do probably better with these AI systems,” says Dr. Hovanesian.
Dr. Armstrong calls AI “a very fancy math equation. These equations have been used for years for various purposes. And now they’re just getting to the point where they can do things that are more sophisticated.”
This tool, adds Dr. Leng, is like any other tool we use in medicine. “This is not going to replace the human physician. It is only going to make us better.” OM
Dr. Armstrong is owner of Ocular Technologies and a consultant to Chart Biopsy, DynaMed, Kriya Therapeutics, Optomed and Xenon-VR. Dr. Leng has no relevant disclosures. Dr. Hovanesian is a consultant to Alcon and Zeiss. Dr. Sheybani is a consultant for AbbVie, Alcon, Glaukos and Nova Eye.