Glaucoma is one of the leading causes of irreversible vision loss worldwide.1 As of 2020, nearly 70 million people have been diagnosed with the disease2,3 — and with an aging population, this figure is expected to increase to over 110 million by 2040.3,4
Diagnosis and follow-up care of glaucoma requires the evaluation of both functional and structural parameters.5,6 The former is assessed with visual field (VF) testing, while the latter relies on optic nerve head (ONH) appearance and quantification of inner retinal thickness layers using optical coherence tomography (OCT).5,6
Identifying damage and subsequent disease progression can be challenging and is often subjective, based on the clinician’s expertise. Clinicians generally rely on trend analyses of functional and anatomical summary metrics (eg, mean and pattern standard deviation for VF testing and global retinal nerve fiber layer [RNFL] thickness on OCT tests), which can be affected by test-retest variability, segmentation errors and the need for several tests before any progression analysis is provided.
In recent years, artificial intelligence (AI) has demonstrated the potential to revolutionize our image-intensive specialty of ophthalmology. AI algorithms have been shown to outperform current standard-of-care methods in the detection and management of several ophthalmic diseases, including glaucoma. This review summarizes the role of AI in glaucoma and its potential benefits in disease detection and progression.
OVERVIEW OF AI TECHNIQUES
How it works
AI algorithms analyze and draw inferences from patterns in data.7 AI comprises a variety of different approaches, including machine learning (ML). Deep learning (DL) algorithms such as convolutional neural networks (CNNs) represent further advancements of ML, capitalizing on the greater computational power available with high performance computing systems.7-11 These biologically inspired algorithms pass input data through a series of nodes, each of which performs computations on data processed by prior nodes. The analysis increases in complexity with each additional layer of analysis; what may be initially detected as a circle by the first few nodes is eventually detected to be a cupped optic nerve by the terminal nodes (Figure).
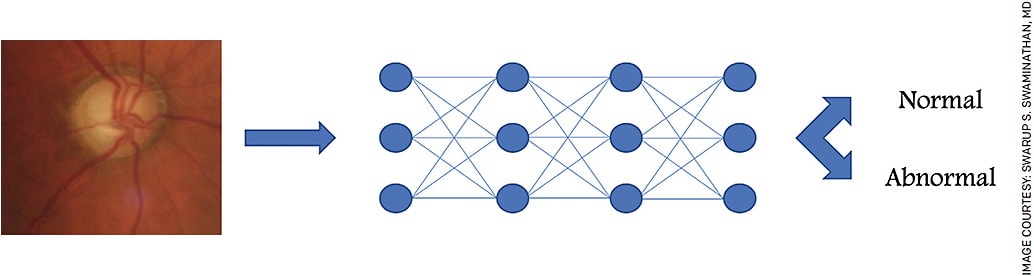
Algorithm training required
To perform a specific task successfully, AI algorithms must be trained on large sets of data, typically on the scale of tens or hundreds of thousands in medicine. This training can be conducted in a supervised, semi-supervised or unsupervised fashion.7-11 These models differ by the amount of labeling applied to the training data. In supervised models, the training dataset is fully labeled with the associated outcome (eg, “glaucoma” or “normal”).
In unsupervised learning, the system must recognize structural patterns without any labels. Semi-supervised learning is a combination of these methods, in which only part of the training set is labeled.
The algorithm works to identify features within the image that are associated with the specific labels. Once trained, AI algorithms require validation to assess the generalizability of the model. This validation dataset is typically derived from a separate database to avoid any bias that could lead to an overestimation of model performance. Accordingly, large datasets are required for reliable AI algorithms — one of the main challenges of this novel technology.
With respect to glaucoma, several AI algorithms have been proposed based on the interpretation of functional (ie, visual fields) and/or structural (ie, fundus photographs and OCT) data.
DIAGNOSING DAMAGE IN GLAUCOMA
Functional damage
DL algorithms have been shown to be particularly effective in discriminating the presence of visual field defects. For example, Li et al developed a DL network trained on visual field pattern deviation maps to distinguish between healthy and glaucomatous tests.12 This algorithm achieved higher accuracy than glaucoma specialists and traditional diagnostic criteria, such as the Advanced Glaucoma Intervention Study and the Glaucoma Staging System 2 criteria. More recently, Kucur et al also developed a similar algorithm, which showed an average precision score of 87%.13
Archetype analysis has also been completed with visual field testing. This analysis is a form of unsupervised machine learning that can identify representative spatial patterns of the grayscale images from visual fields (archetypes), which emphasizes their distinctive features. The VF archetypes defined by the algorithms resemble clinically recognizable patterns of VF loss. Using this approach, several research groups have managed not only to identify a set of VF archetypes, but also to correlate them with demographic characteristics, patterns of RNFL loss and future progression of glaucomatous disease.14-18
The potential of AI as a useful technique in glaucoma management has been demonstrated when evaluating glaucoma progression. Some reports pointed out that AI can identify patterns of progression up to 20 months earlier than traditional methods.19 Wen et al trained a DL network not only to learn spatiotemporal changes of visual fields, but also to forecast future progression up to 5.5 years based on a single baseline test.20
It is important to note that the performances of these approaches are highly variable due to the subjectivity involved in visual field data, which results from changes in patient attention as well as test-retest variability.
In addition, performance of these algorithms is affected by the quality of training sets; these need to be large enough and sufficiently balanced between normal and glaucomatous eyes, as well as in defect severity and location. Finally, the clinician must keep in mind the possibility of misclassification in challenging diagnostic scenarios; patients with tilted or myopic optic nerves often have abnormal visual fields but may not have glaucoma.
Structural damage
Fundus photography and OCT have been the primary structural inputs used to train AI algorithms to detect glaucoma. While the clinical relevance of modalities such as confocal scanning laser ophthalmoscopy has decreased in recent years due to advances in OCT technology, the value of fundus photography has been reestablished by AI. Deep learning analysis of fundus photographs have demonstrated excellent performance in diagnosing glaucoma and in detecting glaucomatous progression.21-23
In a cross-sectional study, Liu et al developed a CNN that classified glaucoma based on fundus photographs with a sensitivity and specificity greater than 95%. Similarly, Li et al developed a DL network trained on 48,000 fundus photographs that could detect glaucomatous cases with a specificity of 92% and a sensitivity of 96%.24 Recently, Medeiros et al were able to train a CNN to predict RNFL thickness using fundus photographs.22 Of note, this DL model was not trained with human labeled fundus photos but rather with RNFL thickness values extracted from OCT.
These types of models could represent a cost-effective strategy for diagnosing glaucoma based on predicted RNFL values using ONH appearance — particularly when visual field and OCT testing are unavailable. This work demonstrated that it is possible to overcome the requirement of human labeling by utilizing other objective sources of information.
Subjectivity and disagreement often occur with human grading, which is a labor-intensive process.
OCT MODALITY: PROS AND CONS
As a highly reproducible image-based diagnostic tool, OCT has been an excellent modality for AI applications in glaucoma. Recent OCT-based DL algorithms were reported to be able to effectively detect glaucomatous ONH changes and disease progression up to 2 years in advance compared to conventional strategies.25-27 OCT provides objective and repeatable quantification of glaucomatous damage by measuring inner retinal layers (RNFL, ganglion cell and inner plexiform layers).
However, segmentation artifacts represent a major challenge to commercially available systems, especially in certain contexts such as severe myopia. Given the need for validation with real-world training datasets, the efficiency and reliability of OCT-based AI approaches can be negatively impacted. Several alternatives have been proposed.
Jammal et al developed a DL algorithm able to detect segmentation errors with a sensitivity of ~99%. Other authors described segmentation-free OCT-based algorithms to provide more accurate predictions of RNFL thickness compared to those obtained by the conventional segmentation-dependent algorithm.8,28,29 Thompson et al showed that the diagnostic performance of a segmentation-free DL algorithm was better than using RNFL thickness metrics, especially in pre-perimetric and mild glaucoma.30 In addition, Mariottoni et al reported that a segmentation-free model performed better than a conventional segmentation-dependent algorithm when evaluating images with errors or other artifacts.31
Other groups proposed the use of unsegmented three-dimensional volumetric maps of the macula and of the optic nerve instead of circle RNFL scans for glaucoma detection, prediction of progression and structure-function correlation.32-35 Both Maetschke et al and George et al showed that class activation maps of their algorithms (heat maps that indicate key aspects of the image used to apply a label) encompassed regions previously established as clinical markers in glaucoma, such as rim area. However, these models also identified retinal layers not traditionally associated with the disease process (eg, retinal pigmented epithelium).33,35
As acknowledged by the authors, these findings show that DL approaches might utilize unexpected features. All these studies suggest that the use of segmentation-free reference datasets could overcome current diagnostic limitations.8,28,29
Finally, DL algorithms have also been trained to create structure-function maps, correlating visual field defects with simulated patterns of RNFL loss.36-38 Such maps could provide predictions of visual field defects corresponding to specific patterns of RNFL damage, improving our understanding of structure-function correlation in glaucoma.
LIMITATIONS IN AI ALGORITHMS
AI algorithms are dependent on the quality of data used for training.39 In order to provide unbiased results, algorithms need to be trained using datasets that are sufficiently large to avoid overfitting and balanced and diverse in terms of age, sex, and race/ethnicity to allow generalizability.40-44 Committees such as Collaborative Community on Ophthalmic Imaging have recommended that data from numerous global centers is needed to create balanced and high-quality datasets for clinical application.39
In contrast to other ocular conditions such as diabetic retinopathy, the lack of a clear standardized, “ground truth” definition of glaucoma makes case labeling and thus model training challenging. Defining true glaucomatous progression on perimetry vs test fluctuation is another challenge. AI may prove to be more useful in identifying the presence of glaucoma rather than in detecting disease progression.
Concerns about the generalizability of AI algorithms are likely to limit their clinical implementation in the near future.
Ultimately, it is unlikely for an FDA-approved glaucoma AI algorithm to be available to clinicians for at least another 5-10 years.
CONCLUSION
AI has the potential to substantially aid clinicians in diagnosing glaucoma and identifying disease progression. While it is unlikely to replace clinicians outright, AI may provide valuable decision support. In addition, it has significant potential to improve eye care in resource-limited settings, potentially serving as a screening tool.
Greater insight into the mechanics of these algorithms, solutions to challenges of data disparities, as well as additional rigorous validation using large, diverse population datasets are warranted prior to clinical implementation. OM
REFERENCES
- G. B. D. Blindness Vision Impairment Collaborators, Vision Loss Expert Group of the Global Burden of Disease Study. Causes of blindness and vision impairment in 2020 and trends over 30 years, and prevalence of avoidable blindness in relation to VISION 2020: the Right to Sight: an analysis for the Global Burden of Disease Study. Lancet Glob Health. Feb 2021;9:e144-e160.
- Zhang N, Wang J, Li Y, Jiang B. Prevalence of primary open angle glaucoma in the last 20 years: a meta-analysis and systematic review. Sci Rep. Jul 2 2021;11:13762.
- Tham YC, Li X, Wong TY, et al. Global prevalence of glaucoma and projections of glaucoma burden through 2040: a systematic review and meta-analysis. Ophthalmology. Nov 2014;121:2081-90.
- Gallo Afflitto G, Aiello F, Cesareo M, Nucci C. Primary open-angle glaucoma prevalence in EUROPE: A systematic review and meta-analysis. J Glaucoma. Aug 9 2022;
- European Glaucoma Society. Terminology and guidelines for Glaucoma. 2021;
- Prum BE, Jr., Rosenberg LF, Gedde SJ, et al. Primary Open-Angle Glaucoma Preferred Practice Pattern((R)) Guidelines. Ophthalmology. Jan 2016;123:P41-P111.
- Kapoor R, Walters SP, Al-Aswad LA. The current state of artificial intelligence in ophthalmology. Surv Ophthalmol. Mar-Apr 2019;64:233-240.
- Salazar H, Misra V, Swaminathan SS. Artificial intelligence and complex statistical modeling in glaucoma diagnosis and management. Curr Opin Ophthalmol. Mar 1 2021;32:105-117.
- Hogarty DT, Mackey DA, Hewitt AW. Current state and future prospects of artificial intelligence in ophthalmology: a review. Clin Exp Ophthalmol. Jan 2019;47:128-139.
- Eslami M, Kim JA, Zhang M, et al. Visual Field Prediction: Evaluating the Clinical Relevance of Deep Learning Models. Ophthalmol Sci. Mar 2023;3:100222.
- Dixit A, Yohannan J, Boland MV. Assessing glaucoma progressionuUsingmMachine learning trained on longitudinal visual field and clinical data. Ophthalmology. Jul 2021;128:1016-1026.
- Li F, Wang Z, Qu G, et al. Automatic differentiation of Glaucoma visual field from non-glaucoma visual filed using deep convolutional neural network. BMC Med Imaging. Oct 4 2018;18:35.
- Kucur SS, Hollo G, Sznitman R. A deep learning approach to automatic detection of early glaucoma from visual fields. PLoS One. Nov. 28, 2018;13:e0206081.
- Wang M, Shen LQ, Pasquale LR, et al. An Artificial Intelligence Approach to Detect Visual Field Progression in Glaucoma Based on Spatial Pattern Analysis. Invest Ophthalmol Vis Sci. Jan 2, 2019;60:365-375.
- Cai S, Elze T, Bex PJ, et al. Clinical correlates of computationallyderived visual field defect archetypes in patients from a glaucoma clinic. Curr Eye Res. Apr 2017;42:568-574.
- Elze T, Pasquale LR, Shen LQ, et al. Patterns of functional vision loss in glaucoma determined with archetypal analysis. J R Soc Interface. Feb 6 2015;12(103)
- Thakur A, Goldbaum M, Yousefi S. Convex Using Deep Archetypal Analysis for Predicting Glaucoma. IEEE J Transl Eng Health Med. 2020;8:3800107.
- Kang JH, Wang M, Frueh L, et al. Cohort Study of Race/Ethnicity and Incident Primary Open-Angle Glaucoma Characterized by Autonomously Determined Visual Field Loss Patterns. Transl Vis Sci Technol. Jul 8 2022;11:21.
- Yousefi S, Kiwaki T, Zheng Y, et al. Detection of Longitudinal Visual Field Progression in Glaucoma Using Machine Learning. Am J Ophthalmol. Sep 2018;193:71-79.
- Wen JC, Lee CS, Keane PA, et al. Forecasting future Humphrey Visual Fields using deep learning. PLoS One. 04/05/2019 2019;14:e0214875.
- Liu H, Li L, Wormstone IM, et al. Development and Validation of a Deep Learning System to Detect Glaucomatous Optic Neuropathy Using Fundus Photographs. JAMA Ophthalmol. Dec 1 2019;137:1353-1360.
- Medeiros FA, Jammal AA, Thompson AC. From Machine to Machine: An OCT-Trained Deep Learning Algorithm for Objective Quantification of Glaucomatous Damage in Fundus Photographs. Ophthalmology. Apr 2019;126:513-521.
- Hood DC, De Moraes CG. Efficacy of a Deep Learning System for Detecting Glaucomatous Optic Neuropathy Based on Color Fundus Photographs. Ophthalmology. Aug 2018;125:1207-1208.
- Li Z, He Y, Keel S, Meng W, Chang RT, He M. Efficacy of a Deep Learning System for Detecting Glaucomatous Optic Neuropathy Based on Color Fundus Photographs. Ophthalmology. Aug 2018;125:1199-1206.
- Christopher M, Belghith A, Weinreb RN, et al. Retinal Nerve Fiber Layer Features Identified by Unsupervised Machine Learning on Optical Coherence Tomography Scans Predict Glaucoma Progression. Invest Ophthalmol Vis Sci. Jun 1 2018;59:2748-2756.
- Belghith A, Bowd C, Weinreb RN, Zangwill LM. A joint estimation detection of Glaucoma progression in 3D spectral domain optical coherence tomography optic nerve head images. Proc SPIE Int Soc Opt Eng. Mar 18 2014;9035:90350O.
- Ratanawongphaibul K, Tsikata E, Zemplenyi M, et al. Earlier Detection of Glaucoma Progression Using High-Density 3-Dimensional Spectral-Domain OCT Optic Nerve Volume Scans. Ophthalmol Glaucoma. Nov-Dec 2021;4:604-616.
- Mursch-Edlmayr AS, Ng WS, Diniz-Filho A, et al. Artificial Intelligence Algorithms to Diagnose Glaucoma and Detect Glaucoma Progression: Translation to Clinical Practice. Transl Vis Sci Technol. Oct 2020;9:55.
- Devalla SK, Liang Z, Pham TH, et al. Glaucoma management in the era of artificial intelligence. Br J Ophthalmol. Mar 2020;104:301-311.
- Thompson AC, Jammal AA, Berchuck SI, Mariottoni EB, Medeiros FA. Assessment of a Segmentation-Free Deep Learning Algorithm for Diagnosing Glaucoma From Optical Coherence Tomography Scans. JAMA Ophthalmol. Apr 1 2020;138:333-339.
- Mariottoni EB, Jammal AA, Urata CN, et al. Quantification of Retinal Nerve Fibre Layer Thickness on Optical Coherence Tomography with a Deep Learning Segmentation-Free Approach. Sci Rep. Jan 15 2020;10:402. doi:10.1038/s41598-019-57196-y
- Russakoff DB, Mannil SS, Oakley JD, et al. A 3D Deep Learning System for Detecting Referable Glaucoma Using Full OCT Macular Cube Scans. Transl Vis Sci Technol. Feb 18 2020;9:12.
- George Y, Antony BJ, Ishikawa H, Wollstein G, Schuman JS, Garnavi R. Attention-Guided 3D-CNN Framework for Glaucoma Detection and Structural-Functional Association Using Volumetric Images. IEEE J Biomed Health Inform. Dec 2020;24:3421-3430.
- An G, Omodaka K, Hashimoto K, et al. Glaucoma Diagnosis with Machine Learning Based on Optical Coherence Tomography and Color Fundus Images. J Healthc Eng. Feb. 18, 2019;2019:4061313.
- Maetschke S, Antony B, Ishikawa H, Wollstein G, Schuman J, Garnavi R. A feature agnostic approach for glaucoma detection in OCT volumes. PLoS One. 07/01/2019 2019;14(7):e0219126.
- Mariottoni EB, Datta S, Dov D, et al. Artificial Intelligence Mapping of Structure to Function in Glaucoma. Transl Vis Sci Technol. Mar 2020;9:19.
- Yu HH, Maetschke SR, Antony BJ, et al. Estimating Global Visual Field Indices in Glaucoma by Combining Macula and Optic Disc OCT Scans Using 3-Dimensional Convolutional Neural Networks. Ophthalmol Glaucoma. Jan-Feb 2021;4:102-112.
- Christopher M, Bowd C, Belghith A, et al. Deep Learning Approaches Predict Glaucomatous Visual Field Damage from OCT Optic Nerve Head En Face Images and Retinal Nerve Fiber Layer Thickness Maps. Ophthalmology. Mar 2020;127:346-356.
- Schuman JS, De Los Angeles Ramos Cadena M, McGee R, et al. A Case for the Use of Artificial Intelligence in Glaucoma Assessment. Ophthalmol Glaucoma. May-Jun 2022;5(3):e3-e13.
- Burlina P, Joshi N, Paul W, et al. Addressing Artificial Intelligence Bias in Retinal Diagnostics. Transl Vis Sci Technol. Feb 5 2021;10:13.
- Lim JS, Hong M, Lam WST, et al. Novel technical and privacy-preserving technology for artificial intelligence in ophthalmology. Curr Opin Ophthalmol. May 1 2022;33:174-187.
- Lin WC, Chen JS, Chiang MF, Hribar MR. Applications of Artificial Intelligence to Electronic Health Record Data in Ophthalmology. Transl Vis Sci Technol. Feb 27 2020;9:13.
- Yang LWY, Ng WY, Foo LL, et al. Deep learning-based natural language processing in ophthalmology: applications, challenges and future directions. Curr Opin Ophthalmol. Sep 1 2021;32:397-405.
- Cheng CY, Soh ZD, Majithia S, et al. Big Data in ophthalmology. Asia Pac J Ophthalmol. Jul-Aug 2020;9:291-298.