Diabetic retinopathy (DR) is a leading cause of blindness worldwide, affecting millions of people with diabetes.1,2 Early detection and timely treatment are crucial for preventing vision loss, but screening programs are often limited by the availability of trained physicians, especially in underserved areas.3,4
In recent years, there has been growing interest in the use of artificial intelligence (AI) for the automated detection of DR.5 AI systems implement machine learning and deep learning techniques to create automated algorithms for DR detection. In this article, we’ll examine the role that various AI technologies play in screening for and detecting DR.
AUTOMATED SCREENING
Overview
Automated DR screening devices are designed to analyze retinal images using AI algorithms to detect signs of DR at an early stage. These devices have the potential to improve the accuracy and efficiency of DR screening by reducing the need for manual interpretation of images, saving time and costs, and allowing for more frequent screening. The use of automated DR screening devices is particularly beneficial in resource-limited settings where access to trained personnel and specialized equipment may be limited.
LumineticsCore
The LumineticsCore AI diagnostic system (formerly IDx-DR) (Digital Diagnostics) was the first autonomous AI-based diagnostic system to receive FDA clearance for commercial use in the United States.6 Using a fundus camera, a trained operator captures two images per eye. These images are submitted to LumineticsCore, where the system analyzes the images for signs of DR. LumineticsCore provides rapid results that health-care providers then review.
Previous studies have evaluated this system in primary care clinics and research trials across different countries.7-9 A study to evaluate the LumineticsCore system included 819 adults with diabetes and no previous DR diagnosis.10 The system correctly identified more than mild diabetic retinopathy (mtmDR) in 173 out of 198 patients, with a sensitivity of 87% and specificity of 90%.10
LumineticsCore system has several benefits, including being easy to learn and generating results within minutes.6 This allows for identification of patients who require referral to a physician during the same visit, which can help reduce referral times in communities where access to specialists is limited. In addition, patients who receive a negative result (ie, no evidence of mtmDR) are advised to repeat testing in 12 months.
Furthermore, LumineticsCore is non-invasive and does not require dilation of the eye. However, there is a risk of missing significant retinopathy if the AI detection system fails as well as a chance for unnecessary referrals due to false-positive results.6
EyeArt
The EyeArt AI eye screening system (Eyenuk Inc.) is another FDA-cleared AI-based system that enables point-of-care screening with a 97% imageability rate for detecting eyes with more than mtmDR.11 To screen for DR, the EyeArt system employs a cloud-based system for analysis following capture of the fundus images using a color retina fundus camera. The system evaluates the images and generates DR screening results. The results are made available for download.
A study was conducted to compare the performance of the EyeArt system with that of retina specialists and general ophthalmologists in detecting mtmDR.12 The results showed that the AI system had higher sensitivity for detecting mtmDR than both specialists and general ophthalmologists. Specifically, the AI system correctly identified 97% of positive cases for mtmDR while the sensitivity for retina specialists was 59.5% and for general ophthalmologists was 20.6%.12 However, the specificity of the AI system was lower compared to both specialists and general ophthalmologists, with 88% correctly identified negative cases for mtmDR.12
One study evaluated the EyeArt system’s capacity to detect eyes with DR that can potentially threaten vision with a sensitivity of 97% and specificity of 90%.11 Also, EyeArt requires fewer (12.6%) patients to be dilated compared to the LumineticsCore system (23.6%) to produce disease detection results.11
Ophthal-360
Ophthal-360 (Ophthalytics) is a camera agnostic system that can detect mild-to-moderate and vision-threatening DR with an associated image quality score. It is still pending FDA clearance. Ophthal-360 is a cloud-based AI eye screening solution that swiftly analyzes retinal images taken with a fundus camera. It efficiently detects any abnormalities present in the images and generates a comprehensive report within seconds of image upload. In addition to evaluating DR, this system has demonstrated feasibility to identify potential diabetic macular edema (DME).
RetmarkerDR software
RetmarkerDR software (Retmarker) is a Class IIa CE-marked medical device that can compare current and previous images to track disease progression and detect microaneurysm turnover rates.13,14 It utilizes a combination of a fundus camera and a laptop equipped with Retmarker. The captured images are securely sent to a designated data center for automatic analysis and diagnosis using the Retmarker Algorithm. A triage team is responsible for conducting analysis and for the grading of relevant cases. The generated report is then made available. One study evaluating RetmarkerDR identified that its sensitivity was 73.0% for any retinopathy, 85.0% for referable retinopathy and 97.9% for proliferative retinopathy when compared to the results of human grading.15
Google DR Algorithm
Google has developed an algorithm based on convolutional neural networks that outperformed human graders in sensitivity for DR and DME in a nationwide screening program in Thailand.16,17 The Thai study analyzed more than 25,000 retinal images from diabetic patients. The algorithm had higher sensitivity (97%) in detecting referable DR compared to human graders (74%), although its specificity was slightly lower (96% vs 98%). The algorithm also exhibited higher sensitivity in identifying severe NPDR, PDR and DME.16
Bosch DR algorithm
Bosch has developed an automatic DR screening solution using the Bosch Mobile Eye Care fundus camera that captures non-mydriatic images. The Bosch DR Algorithm utilizes a deep convolutional neural network to automatically identify DR in fundus images. It has high sensitivity and specificity rates (91%, 97% respectively), but limited information is available about the grading criteria.18
RetinaLyze screening software
RetinaLyze (RetinaLyze System A/S), a Class I device with a CE mark, is a cloud-based software that provides automated screening for DR, AMD and glaucoma.19,20 Image submissions are made through a website-based system, which utilizes end-to-end encryption. In its current web-based form, RetinaLyze includes improvements in deep learning technology. However, there are no recent studies available on its effectiveness in this updated version.
RetinAI
RetinAI has developed a set of Disease Evaluation Apps consisting of CE-marked AI models that are clinically approved for use. These models were trained using real-world evidence and compared to expert graders, demonstrating comparable performance.
A study was conducted on the AI algorithm for automated DR detection. The algorithm was developed using deep learning and was compared to two experienced retinal experts. The results showed that the AI algorithm demonstrated high consistency with the experts, with sensitivity scores of 0.99 and 1, specificity scores of 0.74 and 0.71, and an area under the ROC curve of 0.87 and 0.86 for the observers and algorithm, respectively.21
BEYOND DR ANALYSIS
In addition to DR screening, AI systems have the potential to identify new features that may not be detectable by the human eye. For instance, deep learning algorithms may be capable of incorporating existing complex biomarkers like ultra-widefield fluorescein angiography (UWFA) biomarkers (non-perfusion area, ischemic index, leakage and microaneurysm counts)22,23 (Figure 1) as well as optical coherence tomography biomarkers (central subfield thickness, disorganization of retinal inner layers, hyperreflective foci, ellipsoid zone integrity, retinal fluid index and retinal fluid volatility)24-29 (Figure 2). These biomarkers have shown a correlation with DR severity, and many of these parameters, such as quantitative UWFA parameters, have been strongly associated with DR severity and risk of vision loss.23 Utilizing these systems, both panretinal and zonal vascular features can be extracted. The findings indicated that retinal vasculature features measured with AI systems demonstrated a correlation with DR severity, as well as visual and subvisual vascular biomarkers.30
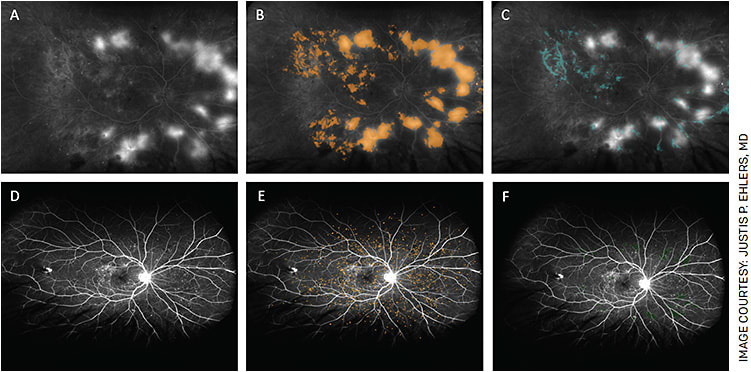
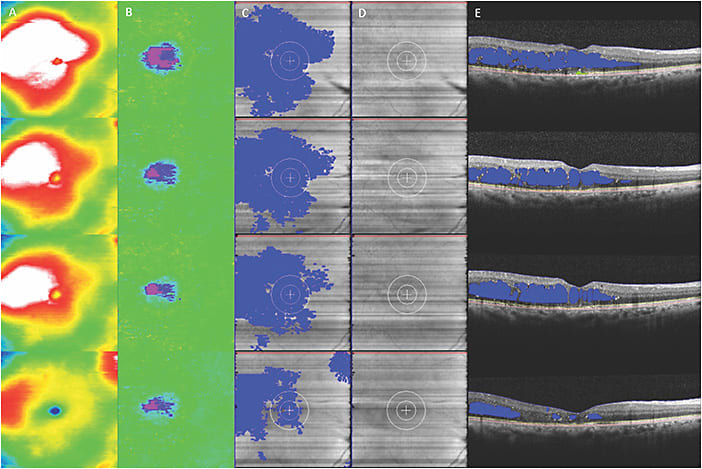
The low quality of fundus images can create uncertainty in diagnosing and grading DR. To overcome this limitation, deep learning models can be utilized to identify critical features of DR on UWFA images. Deep learning models, which were trained and validated using convolutional neural networks for automated segmentation, can accurately detect retinal capillary non-perfusion, neovascularization, disc and outer margins.31 This approach may help to address grader subjectivity in DR diagnosis and grading.
OTHER PROPOSED MODELS
Additional studies have proposed an AI model that combines machine-learning and deep-learning techniques for grading DR with high accuracy. One example, was a system that was trained on 3,543 UWFA images and tested on two separate sets of images, with an overall accuracy of 89.0% and 94.0% for distinguishing between gradable and ungradable images.32 By reducing manual image grading workload and providing almost immediate feedback on image quality during image acquisition, the proposed system has the potential to improve efficiency and accuracy in DR diagnosis through optimizing image acquisition.
Another proposed idea is to enhance low-quality fundus images for DR diagnosis through a collaborative learning framework that jointly trains subnetworks for image quality assessment, enhancement and DR grading.33 This framework models the potential correlation between these tasks and improves both image quality and DR grading performance while preserving structure and lesion features.33
Additionally, AI models have been developed to predict the risk of DR and its progression based on patient characteristics and retinal imaging. One study used an automated microaneurysm detection platform to evaluate changes in microaneurysm counts in DR patients receiving treatment with intravitreal aflibercept injection.34 Findings revealed a significant decrease in microaneurysms in all zones and panretinally in both treatment groups at 6 months and 1 year.34 Therefore, automated microaneurysm detection platforms may provide a novel imaging marker for evaluating disease activity and therapeutic outcomes.
Another study investigated the potential role of UWFA in disease burden assessment, with emphasis on leakage parameters.35 The study found significant improvements in leakage index in eyes treated with intravitreal aflibercept injection in patients with DME.35 This suggests that assessment of UWFA parameters may provide valuable insights into disease progression and treatment efficacy in DME.
AI-based systems could also be used to monitor treatment in DR by identifying predictive biomarkers for a future need for anti-VEGF therapy. A recent study showed that quantitative angiographic features such as macular leakage index, panretinal leakage index, presence of DME and visual acuity were significantly different in eyes requiring immediate or delayed treatment compared to those not requiring treatment in patients with DR.36 A random forest model showed an area under the curve of 0.91, indicating that these features may serve as important predictive biomarkers for improving efficiency of DR treatment and guide follow-up frequency.36
LIMITATIONS AND CHALLENGES
Despite the potential benefits of AI-based automated DR screening devices, there are potential limitations and challenges to their implementation.
For example, the devices and platforms may not be able to generalize across image types, populations or disease subsets based on the underlying training datasets. Variations in image quality and characteristics can affect algorithm performance, as different imaging devices produce images with varying resolution and artifacts. Population differences in demographics and ocular characteristics can also impact algorithm performance.
Also, disease subsets within DR can vary in severity and manifestations, making it difficult for algorithms trained on specific subsets to accurately classify and grade different cases. In addition, there are ethical and regulatory issues related to the use of AI in health care, such as ensuring patient privacy and data security.
CONCLUSION
AI-based automated DR screening devices show promising results in detecting DR and have the potential to improve the efficiency and accuracy of DR screening. These devices have the potential to be implemented in resource-limited settings where access to specialized equipment and trained personnel may be limited. However, further research is needed to evaluate the performance of these devices in different populations and settings.
AI-enabled image feature analysis platforms may provide next-generation assessment of disease burden and risk stratification. With continued advancements in AI technology and imaging, these systems may play an increasingly important role in the early detection, management and risk stratification of diabetic eye disease. OM
REFERENCES
- Fong DS, Aiello L, Gardner TW, et al. Retinopathy in diabetes. Diabetes Care. 2004;27 Suppl 1:S84-S87.
- Leasher JL, Bourne RR, Flaxman SR, et al. Global Estimates on the Number of People Blind or Visually Impaired by Diabetic Retinopathy: A Meta-analysis From 1990 to 2010 [published correction appears in Diabetes Care. 2016 Nov;39(11):2096]. Diabetes Care. 2016;39(9):1643-1649.
- Fundus photographic risk factors for progression of diabetic retinopathy. ETDRS report number 12. Early Treatment Diabetic Retinopathy Study Research Group. Ophthalmology. 1991;98(5 Suppl):823-833.
- Flaxel CJ, Adelman RA, Bailey ST, et al. Diabetic Retinopathy Preferred Practice Pattern [published correction appears in Ophthalmology. 2020 Sep;127(9):1279]. Ophthalmology. 2020;127(1):P66-P145.
- Grzybowski A, Brona P, Lim G, et al. Artificial intelligence for diabetic retinopathy screening: a review [published correction appears in Eye (Lond). 2019 Dec 10;:]. Eye (Lond). 2020;34(3):451-460.
- Savoy M. IDx-DR for Diabetic Retinopathy Screening. Am Fam Physician. 2020;101(5):307-308.
- van der Heijden AA, Abramoff MD, Verbraak F, van Hecke MV, Liem A, Nijpels G. Validation of automated screening for referable diabetic retinopathy with the IDx-DR device in the Hoorn Diabetes Care System. Acta Ophthalmol. 2018;96(1):63-68.
- Abràmoff MD, Folk JC, Han DP, et al. Automated analysis of retinal images for detection of referable diabetic retinopathy. JAMA Ophthalmol. 2013;131(3):351-357.
- Hansen MB, Abràmoff MD, Folk JC, Mathenge W, Bastawrous A, Peto T. Results of Automated Retinal Image Analysis for Detection of Diabetic Retinopathy from the Nakuru Study, Kenya. PLoS One. 2015;10(10):e0139148. Published 2015 Oct 1.
- Abràmoff MD, Lavin PT, Birch M, Shah N, Folk JC. Pivotal trial of an autonomous AI-based diagnostic system for detection of diabetic retinopathy in primary care offices. NPJ Digit Med. 2018;1:39. Published 2018 Aug 28.
- Ipp E, Liljenquist D, Bode B, et al. Pivotal Evaluation of an Artificial Intelligence System for Autonomous Detection of Referrable and Vision-Threatening Diabetic Retinopathy [published correction appears in JAMA Netw Open. 2021 Dec 1;4(12):e2144317]. JAMA Netw Open. 2021;4(11):e2134254. Published 2021 Nov 1.
- Lim JI, Regillo CD, Sadda SR, et al. Artificial Intelligence Detection of Diabetic Retinopathy: Subgroup Comparison of the EyeArt System with Ophthalmologists' Dilated Examinations. Ophthalmol Sci. 2022;3(1):100228. Published 2022 Sep 30.
- Ribeiro L, Oliveira CM, Neves C, Ramos JD, Ferreira H, Cunha-Vaz J. Screening for Diabetic Retinopathy in the Central Region of Portugal. Added Value of Automated 'Disease/No Disease' Grading [published online ahead of print, 2014 Nov 26]. Ophthalmologica. 2014.
- Ribeiro ML, Nunes SG, Cunha-Vaz JG. Microaneurysm turnover at the macula predicts risk of development of clinically significant macular edema in persons with mild nonproliferative diabetic retinopathy. Diabetes Care. 2013;36(5):1254-1259.
- Tufail A, Kapetanakis VV, Salas-Vega S, et al. An observational study to assess if automated diabetic retinopathy image assessment software can replace one or more steps of manual imaging grading and to determine their cost-effectiveness. Health Technol Assess. 2016;20(92):1-72.
- Gulshan V, Peng L, Coram M, et al. Development and Validation of a Deep Learning Algorithm for Detection of Diabetic Retinopathy in Retinal Fundus Photographs. JAMA. 2016;316(22):2402-2410.
- Raumviboonsuk P, Krause J, Chotcomwongse P, et al. Deep learning versus human graders for classifying diabetic retinopathy severity in a nationwide screening program [published correction appears in NPJ Digit Med. 2019 Jul 23;2:68]. NPJ Digit Med. 2019;2:25. Published 2019 Apr 10.
- Bawankar P, Shanbhag N, K SS, et al. Sensitivity and specificity of automated analysis of single-field non-mydriatic fundus photographs by Bosch DR Algorithm-Comparison with mydriatic fundus photography (ETDRS) for screening in undiagnosed diabetic retinopathy. PLoS One. 2017;12(12):e0189854. Published 2017 Dec 27.
- Larsen N, Godt J, Grunkin M, Lund-Andersen H, Larsen M. Automated detection of diabetic retinopathy in a fundus photographic screening population. Invest Ophthalmol Vis Sci. 2003;44(2):767-771.
- Hansen AB, Hartvig NV, Jensen MS, Borch-Johnsen K, Lund-Andersen H, Larsen M. Diabetic retinopathy screening using digital non-mydriatic fundus photography and automated image analysis. Acta Ophthalmol Scand. 2004;82(6):666-672.
- Abreu R, Rodriguez-Martin JN, Donate-Lopez J, et al. Coherence analysis between an artificial intelligence algorithm and human experts in diabetic retinopathy screening. Invest Ophthalmol Vis Sci. 2022;63(7):2110.
- Jiang AC, Srivastava SK, Hu M, et al. Quantitative Ultra-Widefield Angiographic Features and Associations with Diabetic Macular Edema. Ophthalmol Retina. 2020;4(1):49-56.
- Ehlers JP, Jiang AC, Boss JD, et al. Quantitative Ultra-Widefield Angiography and Diabetic Retinopathy Severity: An Assessment of Panretinal Leakage Index, Ischemic Index and Microaneurysm Count. Ophthalmology. 2019;126(11):1527-1532.
- Ehlers JP, Uchida A, Hu M, et al. Higher-Order Assessment of OCT in Diabetic Macular Edema from the VISTA Study: Ellipsoid Zone Dynamics and the Retinal Fluid Index. Ophthalmol Retina. 2019;3(12):1056-1066.
- Ehlers JP, Uchida A, Sevgi DD, et al. Retinal Fluid Volatility Associated With Interval Tolerance and Visual Outcomes in Diabetic Macular Edema in the VISTA Phase III Trial. Am J Ophthalmol. 2021;224:217-227.
- Vujosevic S, Midena E. Retinal layers changes in human preclinical and early clinical diabetic retinopathy support early retinal neuronal and Müller cells alterations. J Diabetes Res. 2013;2013:905058.
- Shi R, Guo Z, Wang F, Li R, Zhao L, Lin R. Alterations in retinal nerve fiber layer thickness in early stages of diabetic retinopathy and potential risk factors. Curr Eye Res. 2018;43(2):244-253.
- Deák GG, Schmidt-Erfurth UM, Jampol LM. Correlation of Central Retinal Thickness and Visual Acuity in Diabetic Macular Edema. JAMA Ophthalmol. 2018;136(11):1215-1216.
- Joltikov KA, Sesi CA, de Castro VM, et al. Disorganization of Retinal Inner Layers (DRIL) and Neuroretinal Dysfunction in Early Diabetic Retinopathy. Invest Ophthalmol Vis Sci. 2018;59(13):5481-5486.
- Sevgi DD, Srivastava SK, Whitney J, et al. Characterization of Ultra-Widefield Angiographic Vascular Features in Diabetic Retinopathy with Automated Severity Classification. Ophthalmol Sci. 2021;1(3):100049.
- Lee PK, Ra H, Baek J. Automated segmentation of ultra-widefield fluorescein angiography of diabetic retinopathy using deep learning [published online ahead of print, 2022 Oct 14]. Br J Ophthalmol. 2022;bjophthalmol-2022-321063.
- Li HH, Abraham JR, Sevgi DD, et al. Automated Quality Assessment and Image Selection of Ultra-Widefield Fluorescein Angiography Images through Deep Learning. Transl Vis Sci Technol. 2020;9(2):52. Published 2020 Sep 17.
- Hou Q, Cao P, Jia L, Chen L, Yang J, Zaiane OR. Image Quality Assessment Guided Collaborative Learning of Image Enhancement and Classification for Diabetic Retinopathy Grading [published online ahead of print, 2022 Dec 23]. IEEE J Biomed Health Inform. 2022;PP:10.1109/JBHI.2022.3231276.
- Babiuch A, Wykoff CC, Hach J, et al. Longitudinal panretinal microaneurysm dynamics on ultra-widefield fluorescein angiography in eyes treated with intravitreal aflibercept for proliferative diabetic retinopathy in the recovery study. Br J Ophthalmol. 2021;105(8):1111-1115.
- Sarici K, Yordi S, Martin A, et al. Longitudinal Quantitative Ultrawide-field Fluorescein Angiography Dynamics in the RUBY Diabetic Macular Edema Study [published online ahead of print, 2023 Feb 2]. Ophthalmol Retina. 2023;S2468-6530(23)00037-4.
- Jiang AC, Sevgi DD, Mugnaini C, et al. Predictive Assessment of Quantitative Ultra-Widefield Angiographic Features for Future Need for Anti-VEGF Therapy in Diabetic Eye Disease. J Pers Med. 2022;12(4):608. Published 2022 Apr 10.