While the buzz surrounding “artificial intelligence” (AI) has increased across medicine in recent years, its origins date back nearly 70 years. First conceptualized in the 1950s, AI is a broad term that describes the science of making computer programs that can imitate human intelligence and critical thinking.1 AI applications in medicine began to appear in the 1970s, with the first Artificial Intelligence in Medicine workshop held in 1975.2
With the rapid increase in computing power and availability of large datasets, AI has expanded across numerous medical fields, including ophthalmology, radiology and oncology. In this article, we introduce some recent applications of AI in the field of glaucoma.
EARLY AND BROAD APPLICATIONS
Ophthalmology is a field especially conducive to development of AI algorithms due to the central role of diagnostic testing and imaging in patient care. One of the first applications of AI in medicine was a glaucoma consultation program developed in 1976.2
In the past decade, AI has been broadly applied in glaucoma to increase patient access to care and enhance provider diagnostic capabilities. AI has also expanded into the fields of retina and cornea, where it holds similar promise for modernizing clinical care.
FUNDUS PHOTO EVALUATION
Fundus photos have been highly popular for developing deep learning (DL) algorithms due to their accessibility and relative simplicity compared to other imaging modalities. DL algorithms trained on ophthalmologist-graded fundus photos have been developed to detect glaucomatous optic neuropathy (GON) in fundus photos.3 With just one fundus photo, these algorithms can detect glaucoma with performance comparable to an ophthalmologist with access to other diagnostic tests.4
A recent meta-analysis comparing DL algorithms to ophthalmologists in identifying GON from fundus photos showed that the performances of the worst DL algorithms and the best ophthalmologists were similar.5 DLalgorithms have also been able to accurately predict retinal nerve fiber layer (RNFL) thickness and identify progressive thinning from fundus photos.6,7
SOME DEFINITIONS
Artificial intelligence (AI): The science of making computer programs that can imitate human intelligence and critical thinking.
Machine learning (ML): A subfield of AI commonly applied in data analysis to predict outcomes. ML uses data that are partly based on patterns that humans have already identified.
Deep learning (DL): A subfield of machine learning that can auto-detect patterns in data to predict outcomes, sometimes identifying patterns that may not be apparent to humans.
These developments are promising for telemedicine and expanding glaucoma screening efforts, especially in geographic regions with limited access to eye care. DL algorithms can also support clinical decision-making by providing glaucoma expert-level evaluations of the optic nerve head (ONH). However, challenges persist in differentiating between myopic and glaucomatous ONHs, as well as optic nerve changes secondary to non-glaucomatous optic neuropathies.
AUTOMATED DETECTION OF GLAUCOMA RISK FACTORS
AI can also be applied to detect risk factors for glaucoma. This includes angle closure, which contributes to approximately a quarter of all cases of glaucoma worldwide.8 Early detection of angle closure is important, because effective laser and surgical treatments are available to alleviate angle closure and reduce glaucoma risk in at-risk eyes.9
Manual gonioscopy is the current clinical standard for evaluating the anterior chamber angle and detecting patients with angle closure. Two general approaches have been used to detect gonioscopic angle closure using anterior segment OCT (AS-OCT) imaging: image-based and measurement-based. AS-OCT images can be directly analyzed using image-based DL algorithms to detect gonioscopic angle closure.10,11
Alternatively, measurements of anatomical risk factors for angle closure can be taken from AS-OCT images and used to develop measurement-based machine learning (ML) algorithms to detect gonioscopic angle closure.12,13
The resulting algorithms could assist in screening for angle closure in cases where providers are not experienced or comfortable with performing gonioscopy. Gonioscopy has also been shown to correlate poorly with angle width measurements obtained from AS-OCT; therefore, AS-OCT could provide objective and quantitative data to supplement gonioscopy.14
The scleral spur is a key anatomical landmark that must be identified in AS-OCT images to obtain measurements of important biometric parameters in angle closure (Figure 1). Unfortunately, scleral spur detection is an expertise-dependent and time-consuming task that requires manual labeling. However, DL algorithms that provide expert-level detection of the scleral spur in AS-OCT scans have recently been developed, which could greatly enhance the clinical utility of AS-OCT.15
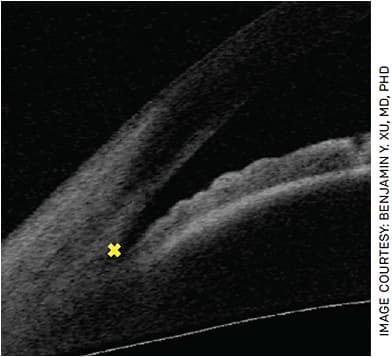
QUESTIONS AND TRADEOFFS
The two approaches to assessing angle closure from AS-OCT (image-based and measurement-based) demonstrate different ways AI can be applied to achieve the same goal. In the image-based approach the DL algorithm auto-detects patterns to use in its predictions, whereas in the measurement-based approach the ML algorithm uses patterns selected by humans. It can be difficult to compare the performance of these different algorithms, as each is developed using different datasets and types of data.
This begs the question: Do algorithms developed without a priori knowledge of biologic significance of image features perform better than algorithms developed using human-selected parameters of known disease risk factors? A study comparing the two methods using the same dataset found that the image-based DL algorithm was better at predicting angle closure than the measurement-based ML algorithm.16 In this case, with the authors’ specific choice of biometric parameters, the DL algorithm was superior.
While this doesn’t answer the question of whether just the right combination of parameters would have improved performance, it does bring us to one of the tradeoffs of AI-based methods: explainability vs performance.17 DL algorithms often provide excellent performance at the cost of low explainability; that is, it is not immediately obvious which features in the data the algorithm uses to make its predictions. Saliency maps can show us which pixels are important to the algorithm’s decision-making (Figure 2). These generally overlap with regions that human graders assess when detecting angle closure.
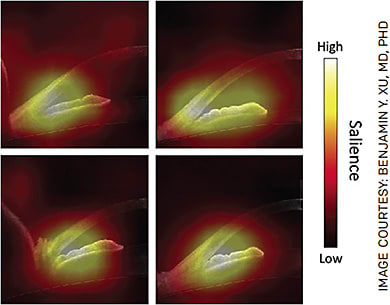
However, the maps also show involvement of other regions that humans may not find relevant but boost the algorithm’s accuracy. ML algorithms, on the other hand, often provide better explainability at the cost of lower performance; it is easier to determine the relative importance of human-selected parameters even if the algorithm’s predictions are not as accurate.
FUTURE DIRECTIONS AND LIMITATIONS
Many challenges remain in balancing the explainability and performance of AI algorithms. Explainability affects how much eye-care providers can trust DL algorithms to help make clinical decisions.
Another limitation faced by AI algorithms is “generalizability.” That is, before an algorithm can be widely adopted for clinical use, it must demonstrate robust performance in diverse populations. This step requires additional data and potentially further refinement of the AIalgorithm.
Finally, AI algorithms typically benefit from wider adoption of imaging technologies. In the case of AS-OCT for angle-closure screening, this would require broader adoption of AS-OCT devices, incorporation of these devices into clinic workflows, and training of technicians and eye-care providers to operate the machines and interpret the results.
As AI evolves, it presents exciting opportunities for expanding screening services and enhancing diagnostic and decision-making capabilities in all fields of ophthalmology. Automated AI algorithms could help eye-care providers incorporate and analyze data in a more convenient and efficient manner, such as assessing RNFL data from an entire OCT cube rather than through a single circular cross-section.18 They could also offer objective data for evaluating patients for glaucoma risk factors, such as angle closure, that currently rely on more subjective or expertise-dependent assessments. OM
REFERENCES
- McCarthy J. What is AI? / Basic questions. http://jmc.stanford.edu/artificial-intelligence/what-is-ai/index.html . Accessed Feb. 2, 2022.
- Kaul V, Enslin S, Gross SA. History of artificial intelligence in medicine. Gastrointest Endosc. 2020;92:807-812.
- Shibata N, Tanito M, Mitsuhashi K, et al. Development of a deep residual learning algorithm to screen for glaucoma from fundus photography.
- Phene S, Carter Dunn R, Hammel N, et al. Deep learning and glaucoma specialists: The relative importance of optic disc features to predict glaucoma referral in fundus photographs. Ophthalmology. 2019;126:1627-1639.
- Buisson M, Navel V, Labbé A, et al. Deep learning versus ophthalmologists for screening for glaucoma on fundus examination: A systematic review and meta-analysis. Clin Exp Ophthalmol. Published online December 2021.
- Medeiros FA, Jammal AA, Thompson AC. From machine to machine: An OCT-trained deep learning algorithm for objective quantification of glaucomatous damage in fundus photographs. Ophthalmology. 2019;126:513-521.
- Medeiros FA, Jammal AA, Mariottoni EB. Detection of progressive glaucomatous optic nerve damage on fundus photographs with deep learning. Ophthalmology. 2021;128:383-392.
- Quigley H, Broman AT. The number of people with glaucoma worldwide in 2010 and 2020. Br J Ophthalmol. 2006;90:262-267.
- He M, Jiang Y, Huang S, et al. Laser peripheral iridotomy for the prevention of angle closure: a single-centre, randomised controlled trial. Lancet. 2019;393(10181):1609-1618.
- Randhawa J, Chiang M, Porporato N, et al. Generalisability and performance of an OCT-based deep learning classifier for community-based and hospital-based detection of gonioscopic angle closure. Br J Ophthalmol. Published online October 20, 2021:bjophthalmol-2021-319470.
- Xu BY, Chiang M, Chaudhary S, Kulkarni S, Pardeshi AA, Varma R. Deep learning classifiers for automated detection of gonioscopic angle closure based on anterior segment OCT images. Am J Ophthalmol. 2019;208:273-280.
- Foo LL, Nongpiur ME, Allen JC, et al. Determinants of angle width in Chinese Singaporeans. Ophthalmology. 2012;119:278-282.
- Nongpiur ME, Haaland BA, Perera SA, et al. Development of a score and probability estimate for detecting angle closure based on anterior segment optical coherence tomography. Am J Ophthalmol. 2014;157:32-38.e1.
- Xu BY, Pardeshi AA, Burkemper B, et al. Differences in anterior chamber angle assessments between gonioscopy, eyecam, and anterior segment OCT: The Chinese American eye study. Transl Vis Sci Technol. 2019;8:5.
- Xu BY, Chiang M, Pardeshi AA, Moghimi S, Varma R. Deep neural network for scleral spur detection in anterior segment OCT images: The Chinese American Eye Study. Transl Vis Sci Technol. 2020;9:1-10.
- Fu H, Baskaran M, Xu Y, et al. A deep learning system for automated angle-closure detection in anterior segment optical coherence tomography images. Am J Ophthalmol. 2019;203:37-45.
- Thompson AC, Jammal AA, Medeiros FA. A review of deep learning for screening, diagnosis, and detection of glaucoma progression. Transl Vis Sci Technol. 2020;9:1-19. https://www.ncbi.nlm.nih.gov/pmc/articles/PMC7424906 . Accessed Feb. 8, 2022.
- Richter GM, Sylvester B, Chu Z, et al. Peripapillary microvasculature in the retinal nerve fiber layer in glaucoma by optical coherence tomography angiography: Focal structural and functional correlations and diagnostic performance. Clin Ophthalmol. 2018;12: 2285-2296.