Artificial intelligence (AI) “is a big deal for all of medicine and ophthalmology, and it is front and center because the engine that drives AI is data, and one of the best sources of data is imaging,” says Srinivas R. Sadda, MD, president and chief scientific officer of the Doheny Eye Institute at the University of California Los Angeles. “We can capture images of the eye with a variety of different methods, and that’s what makes ophthalmology well positioned to be at the vanguard, if you will, of AI and deep learning.”
AI “will help us clinicians … integrate more evidence and more clinical and imaging data into our diagnostic and management decisions,” agrees Michael Abramoff, MD, PhD, founder and CEO of IDx, a company devoted to developing AI-based eye-care technology.
“AI will really take a lot of burden off the ophthalmologist,” says Uday Devgan, MD, a principal in Advanced Euclidean Solutions, developers of an AI-based online tool aimed at increasing the accuracy of IOL calculations.
GOING DEEP IN DR AND MACULAR EDEMA
A study published last year in the British Journal of Ophthalmology (BJO) analyzed AI and deep learning with respect to their benefits for ophthalmology. The study, titled “Artificial intelligence and deep learning in ophthalmology,” found that AI based on deep learning is “achieving robust classification performance” in a variety of eye conditions.
According to Data Science Central (datasciencecentral.com ), deep learning, as well as its close relative, machine learning, are both subsets of AI. Machine learning focuses on the ability of machines to receive a set of data and learn for themselves, changing algorithms as they learn more about the information they are processing. Deep learning, also referred to as “deep neural networks,” in turn, is a subset of machine learning in which “machines,” such as imaging technologies, are exposed to large quantities of items, or data, to be trained in identifying any given object or, in the case of ophthalmology, condition or disease.
An early example of this, according to Data Science Central, was Google Brain learning to recognize cats based on its exposure to 10 million images.
“Diabetic retinopathy (DR) is certainly the lowest-hanging fruit, for obvious reasons,” says Dr. Abramoff, who was not involved in the BJO study, citing “extensive scientific evidence for the benefits of early DR diagnosis, timely treatment and widely agreed upon clinical surrogate outcome markers for this chronic disease.”
IDx received FDA marketing clearance in 2018 for IDx-DR, the first “autonomous” AI-based diagnostic solution for DR authorized by the FDA for commercialization (Figure 1). This technology can be used to screen for and diagnose DR, as well as center-involved and clinically significant macular edema, without requiring a physician’s interpretation (see “A CPT code is in sight”). It is currently in use at dozens of clinics in the United States and the European Union and has provided a diagnostic assessment for thousands of patients, according to the company.
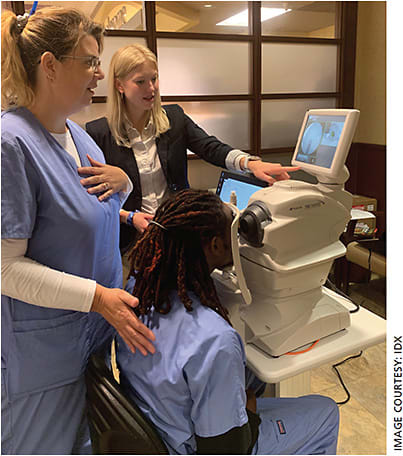
At last October’s annual AAO meeting, Dr. Sadda presented a paper on Eyenuk’s proposed EyeArt AI Eye Screening System for DR (Figure 2). A study of the system, published in the peer-reviewed journal Diabetes Technology & Therapeutics, found that the EyeArt system achieved greater than 91% sensitivity and specificity for detection of referable DR. Eyenuk is currently awaiting a decision from the FDA on its application for marketing approval of EyeArt.
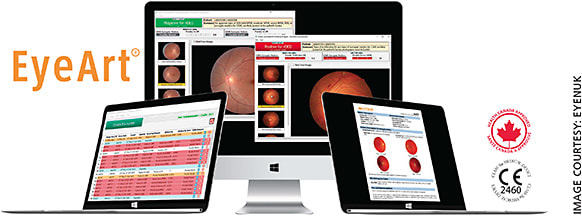
“There is an epidemic of diabetes worldwide, and it definitely makes sense for DR to be the first big target, especially since 90% of blindness from DR is preventable with early detection and proper management,” agrees Dr. Sadda.
OTHER CONDITIONS
But DR and macular edema are just two of the more visible targets of AI’s growing potential to transform eye care. Various companies also have solutions for other eye conditions in their sights.
“People need to be aware it’s just the beginning and that we’ll be screening for other eye conditions — the big ones like glaucoma, like macular generation,” says Dr. Sadda.
Researchers are also working on applications for AMD, glaucoma screening and retinopathy of prematurity. “Generally speaking, the investment in safe, efficient and equitable AI is especially warranted when the condition has high prevalence, there is scientific evidence that early diagnosis can improve outcomes and there are agreed-upon standards for quantifying outcome,” Dr. Abramoff explains.
CATARACT SURGERY WILL BENEFIT, TOO
Cataracts appear to be another such example. Cataract surgery is the most commonly performed procedure worldwide, and AI is being leveraged to improve the accuracy of IOL calculations and provide these patients with sharper vision than can be achieved with current formulas and technologies alone, says Dr. Devgan. He is a codeveloper of IOLcalc.com , a free, AI-based, online calculator that he says can produce far more accurate IOL lens calculations than those generated by current formulas.
“Historically, we’ve been pretty bad with lens calculations,” Dr. Devgan says, explaining that the UK’s Royal College of Ophthalmologists calls for 60% accuracy for lens calculations, while the best U.S. ophthalmologists can achieve 80% to 82% accuracy. “We have a way of doing it with AI that’s easily 90%, 94%, 95% accurate.”
FUTURE GOALS: DIAGNOSIS, PROGNOSIS
Technologies based on AI also show promise in serving as “physician assist” tools to aid diagnosis and improve treatment decisions.
“It may be that the most junior ophthalmologist doesn’t have the expertise of someone who has been in practice for 30 years, but AI has the potential to bring some of that expertise because it can be used to capture the expertise of many people,” explains Dr. Sadda. “Screening is all about answering the question, ‘Is something there, or not?’ A diagnosis answers the question, ‘What is it?’ The next big step for AI is to determine the diagnosis.”
He cites ongoing research into AI-based algorithms that can calculate the probability of any given finding being diagnosed as AMD, DR or another condition based on OCT scans. “Say you’re very busy, seeing a lot of patients, and you come across a patient where you’re not sure of the diagnosis. You might run an AI tool that’s been trained on 1,000 cases of this condition and 1,000 cases of that condition. It might come back with an 80% probability that it’s one condition vs. another condition,” he says. “Of course, as the clinician, you’d have to evaluate that information and make sure it makes sense, but you could proceed from there.”
Yet another aspect of eye care being targeted by researchers relates to AI’s potential utility as a prognostic tool. Emerging data show AI can be used to prognosticate the outcome of a given case based on imaging and other data, then to recommend the best treatment. Once perfected, such capability could further the cause of personalized medicine.
A CPT code is in sight
As of January 2021, IDx’s IDx-DR, an autonomous AI system that detects diabetic retinopathy, will have an AMA current procedural code to facilitate correct billing of the diagnostic. “By accepting this new CPT code, the AMA’s CPT editorial panel has established a billing code for an AI-enabled system that can help foster further adoption of autonomous AI technologies in health care,” Dr. Abramoff said in a company press release. “This will make billing the IDx-DR exam more straightforward for our customers, who have been billing for this sight-saving exam.”
The current code description, “for automated point-of-care retinal imaging,” may be refined prior to its implementation, the company warned.
“Clinical trials are based on hundreds, even thousands of patients,” Dr. Sadda says. “Yes, en masse, a certain outcome can be expected, but … there’s a spread of patients who do worse and patients who do better. The idea with AI is that if you’re able to provide enough training data, AI can figure out who’s going to do better and who’s going to do worse” and enable a more targeted or personalized treatment.
The AAO’s IRIS Registry database is already being utilized for this purpose.
CHALLENGES STILL LIE AHEAD
Nonetheless, AI is not without its challenges and risks. According to the latest available data from the AAO, the current U.S. population of ophthalmologists stands at about 19,200, and technology that can expand patient access to care, while a positive development, also is expected to increase demand for eye-care services, explains Dr. Sadda.
Noting that 20 million patients with diabetes alone require DR screening, he observes that “We’re going to have to be able handle all of these individuals identified with these screening approaches. Some communities, obviously, have a lot of specialists. But, in others, people must travel long distances to see a specialist, and some may not have the means to see a specialist.”
Other challenges revolve around such issues as explainability of AI algorithm results, medicolegal concerns, physician and patient acceptance of AI, coding and reimbursement. Quality-measure guidelines that weren’t needed prior to the advent of AI must also be addressed, says Dr. Abramoff.
“One by one, we have been addressing these. The path forward is becoming clearer,” he says. Still, he cautions against overselling the potential of AI.
“The public, and even physicians, have genuine concerns about AI: Does it actually improve patient outcomes? Is it safe? Is it validated against the right standard? Is the patient-derived training data stewarded? Where is the liability? It is crucial that we discuss the risks and benefits openly, with accountability and transparency about all these aspects,” Dr. Abramoff explains.
Among them, AI systems are only as good as the data used to train them, and the reasoning behind their results — which may in fact be accurate — aren’t always fully understood, potentially leading to unforeseen consequences. Dr. Sadda cites the example of a system that could distinguish a Siberian husky from a golden retriever. When the system was interrogated for its reasoning, researchers determined that all the pictures of the husky had snow in the background.
“The AI wasn’t making the decision based on the dog itself. It was making the decision based on the background,” he says, noting that this same danger, known as “overfitting,” exists in training AI to distinguish various eye conditions, such as tumors.
“Maybe all of the examples of a certain tumor that you give the system also happen to have some other finding present. The AI system learns that that other finding is critical to making this diagnosis, but it turns out it doesn’t have anything to do with the disease,” he says. The goal, Dr. Sadda adds, should be to develop AI solutions that can generalize their training to specific cases just enough to make accurate diagnoses.
OPHTHALMOLOGISTS WILL KEEP THEIR JOBS
As for the risk of AI replacing ophthalmologists, well, that doesn’t appear to be in danger of occurring any time soon.
“Ten years ago, a common fear with AI was that it will displace jobs, and physicians are not exempt from this fear,” says Dr. Abramoff. “But, I think that by being open about the how and why of AI and its benefits and risks, ophthalmologists are now in fact excited.”
Dr. Sadda agrees but notes, “These systems will always be only as good as their training. They can get fooled, and that’s where you always want to have physician oversight.” OM